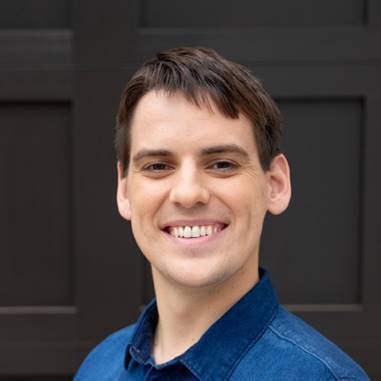
The costs for drug discovery and development are skyrocketing, but AI (artificial intelligence) is introducing new efficiencies to help find effective treatments faster.
A study in 2020 concluded that the estimated median capitalized research and development cost per product was $985 million. Contributing factors to this included larger trial sizes, the need to assess health technology, the requirement to provide data on comparative drugs’ effectiveness, and most importantly the high failure rate. Seven out of eight compounds that enter the clinical testing pipeline are never developed.
So where does AI come in? AI can speed up the processes of collecting and accessing information to drastically shorten drug development time to keep the price of drug development in check. The proportion of pharmaceutical companies hiring for AI-related positions kept relatively steady in May 2022 compared with the equivalent month last year, according to a Pharmaceutical Technology study, with 39.3% of the companies included in the analysis recruiting for at least one such position.
AI Success Stories
Combining information from multiple sources to draw better conclusions faster, AI has the potential to find previously unexplored patterns not immediately obvious to the human eye which can lead to a new understanding of diseases and the drugs designed to treat them.
AI has successfully accelerated drug development for a wide variety of treatments from slowing the aging process to treating rare diseases.
Here are just a few examples:
– Astra Zeneca uses machine learning models to analyse the imaging-based outputs of CRISPR screen in order to discover more quickly which genes can cause resistance to cancer treatments
– Novartis uses machine learning to identify patient groups and predict their positive and adverse reactions to treatments
– Samsung reduces the time required to label lung X-Rays used to diagnose COVID-19 by 84% by using AI to identify and then label the most informative images first.
– Exscientia LTD has developed the world’s first Alzheimer’s disease drug candidate designed by AI.
– Taisho Pharmaceutical of Japan and Insilico Medicine, a Hong Kong-based AI startup, started a joint research project to identify therapeutic compounds that may slow the cellular effects of aging.
– Cyclica has partnered with Bayer to create an AI-augmented integrated network of cloud-based technologies, that identifies significant protein targets, determines the drug’s effect on these targets. and produces a visual output of how the drug and proteins to determine new uses for existing drugs.
Operational Challenges of AI
Despite the high number of successes, there are unique challenges that need to be overcome for pharmaceutical (pharma) companies to reap the benefits of AI. Data is more highly varied and complex which makes building an algorithm that can generate reliable insights more challenging. Pharma data sets are usually smaller with fewer patients and fewer observations per patient. At the same time, there are far more features for each dataset including different graphical formats such as medical images.
Data labeling is more complex and requires highly specialized input, for example, each image of the brain used for cancer screening needs to be inspected by three doctors and each inspection can take 15 minutes.The pharma industry is also highly regulated, requiring full disclosure and transparency for each stage of the process which requires more man-hours and costs for documentation.
In addition to these challenges specific to their industry, pharma companies are all also presented with classic barriers to implementation. For example, the need for a flexible infrastructure to collect data, verify data, run applications, and perform data governance. There is also the need to scale to manage fast-growing data volumes, support more features for each data set, and add more users as more employees within the organization need fast access to data.
Along with these challenges, there has been a corresponding wave of innovation designed to simplify the process of designing and deploying AI/machine learning models. There are new tools and technologies available including pre-built machine learning models that are fully trained and MLOps platforms that can not only help train models but also streamline the process of moving machine learning models into production.
The pharma industry is successfully leveraging AI to speed up data discovery and development. The process of identifying potential treatments, predicting reactions to drugs, and identifying the relevant patients for clinical trials, as well as analyzing medical data to better diagnose diseases and find potential cures had been accelerated while minimizing costs.
The heightened competition to find cures faster will increase the demand to accelerate new drug discoveries. The challenges of analyzing medical data are becoming more complex but so are the capabilities of AI tools and technologies to uncover wiser insights faster.
About Michael Galarnyk
Michael Galarnyk works in Developer Relations and is the ML and AI Evangelist at cnvrg.io, an Intel company. In his spare time, he teaches Python based Machine Learning classes through Stanford Continuing Studies and LinkedIn Learning. You can find him on Twitter, Medium, and GitHub.
About cnvrg.io
cnvrg.io is an AI OS, transforming the way enterprises manage, scale and accelerate AI and data science development from research to production. The code-first platform is built by data scientists, for data scientists and offers unrivaled flexibility to run on-premise or cloud. From advanced MLOps to continual learning, cnvrg.io brings top of the line technology to data science teams so they can spend less time on DevOps and focus on the real magic – algorithms.