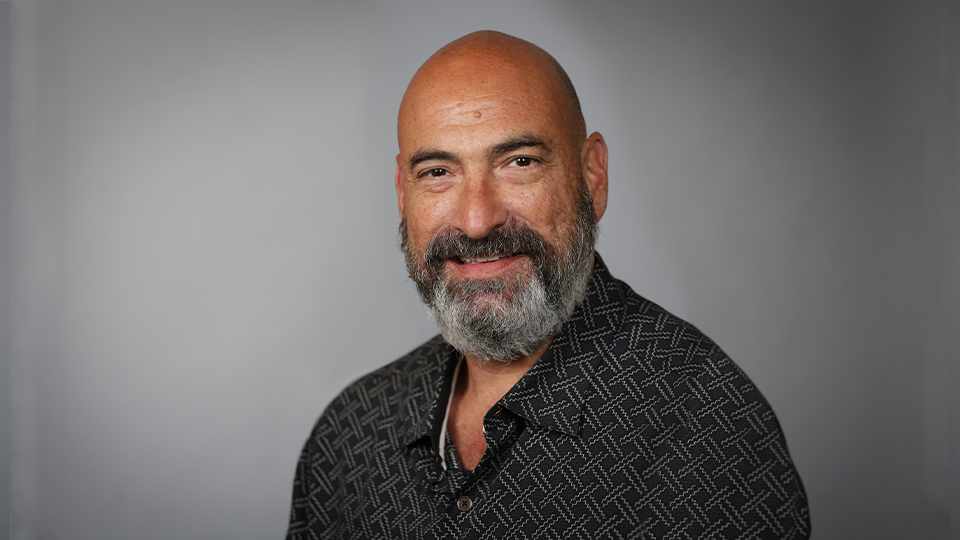
The saying “you cannot manage what you cannot measure” applies to all aspects of healthcare delivery, but it is especially true in value-based care. After all, payers, providers, and the patients they care for need to know what high-value care looks like at an individual level – and how it can be applied at the population level.
Delivery and technology infrastructure. All too often, data is captured in disparate systems, forcing administrative staff – or, worse, physicians and nurses – to find the right diagnostic and billing codes that apply to each episode of care. It’s no wonder that this leads to further frustration from overburdened staff who are forced to enter the same information in many places in multiple formats simply to meet reporting requirements.
This does not have to be the case. With the right technology operating behind the scenes, provider organizations can “translate” clinical documentation into the proper data format for various administrative needs, saving time and resources while at the same time improving the accuracy of those codes captured. Organizations also benefit from a normalized data set that can be readily analyzed, allowing for data-driven decision support at the point of care as well as more informed business planning. When this happens, data and technology evolve from an obstacle to an enabler of value-based care.
Value-based care relies on data
Whether organizations participate in government programs or create value-based care models in partnership with payers, they need to demonstrate that they are in fact providing high-value care. This requires tracking a wide range of metrics: Improved outcomes, lower costs, fewer barriers to care access, improved efficiency, higher patient and clinician satisfaction, and so on.
This data serves two key purposes. It measures an organization’s performance against its value-based care contract. It also allows providers and payers to better understand where high-value care is most readily available and how the best practices for that clinician or facility can be applied across the broader continuum of care.
Admittedly, this is a tall order. It requires organizations to describe a patient accurately from the very beginning of their relationship with a provider and to update that clinical description over time. Otherwise, their knowledge of a patient’s condition, the care they have received, and the clinical pathways that have been recommended for them will be inaccurate – and so, too, will be the measures of whether value-based care has been provided.
Data’s role in value-based medicine has been limited
Despite the clear value proposition for gathering data at the point of care, most provider organizations have been slow to do so at a large scale. There tend to be five reasons for this reluctance.
Competing priorities. Given the need to demonstrate performance for the sake of reimbursement, many providers focus their attention on downstream quality reporting. Similarly, they may be looking for additional opportunities to generate revenue, within a value-based care contract (such as preventive care), from traditional fee-for-service setups, or through work such as clinical trial enrollment. Each of these priorities can push data efforts onto the back burner.
Data format discrepancies. Performance measurement relies on data in structured formats such as those found in ICD-10 and SNOMED code sets. Data captured at the point of care is often unstructured clinical documentation. Expecting clinical staff to document for performance measurement not only distracts from the practice of medicine but also strips the specificity from notes. Patients can be described in many unique and valuable ways in written notes, but usually in vague or abstract ways using diagnostic and billing codes.
Complex workflows. Far too many clinicians feel they are asked to do too much in not enough time. Anything that slows down their work and takes their attention from patient care is a non-starter. This includes additional data entry, especially in code sets with which they are not familiar. It may also include clinical decision support that is presented at the wrong time in a workflow or in a separate application, forcing clinical staff to retrace their steps or close their current window.
Additional data sources. The information that gives clinicians a longitudinal view of the patients they see increasingly comes from beyond the electronic health record (EHR). Some come from external providers such as urgent care clinics or telehealth services. Some come from an organization’s operational and financial business units. As these data sources are often siloed, and therefore difficult to extract and normalize data for the purposes of prospective analysis, they often remain unused.
High variability. Despite long-standing evidence-based best practices, care delivery is highly variable; two doctors seeing the same patient with the same condition in the same clinic at the same time may come up with two different treatment plans. Both of which may be correct for that patient. Providers must also contend with variabilities in cost (as two treatment options with similar outcomes can vary by an order of magnitude in cost) and in insurance plan types (resulting in dozens of workflows for referrals or prior authorization). Altogether, this makes it difficult for organizations to create consistent best practices for gathering and normalizing data.
How the right dose of AI can support value-based care
For some clinicians, the term artificial intelligence conjures images of computers making diagnostic decisions. In practice, AI is not replacing the clinician’s extensive training, hands-on experience, and empathy for patients in need. Rather, AI is supporting the important work they do by streamlining tasks that are traditionally labor-intensive and time-consuming. These tasks include:
– Aggregating and analyzing data from more sources in less time, including valuable data that originated outside the four walls of the provider setting.
– Using natural language processing to “translate” clinical documentation into ICD-10, SNOMED, and other relevant operational and administrative codes.
– Automating workflows for collecting data at the point of care as well as submitting that data for financial and quality reporting processes.
Through these processes of aggregation, translation, and automation, AI is positioned to enhance value-based care in five important ways.
– Leveraging AI in the background to generate the right diagnostic and billing codes preserves the highly nuanced clinical information captured at the point of care. In addition, patient visits are no longer disrupted while clinicians scroll or search for the appropriate codes.
– Clinicians are free to write their notes using the medical terminology they are used to, providing a level of specificity and personalization that medical coding and claims data cannot capture.
– Normalization of data from disparate sources provides a longitudinal view of patient health and well-being that is no longer limited to what has been captured within the EHR.
– AI can surface data and evidence-based insight within the EHR at the appropriate point in the workflow when clinicians are making decisions that impact variability in care pathways and costs for individual patients.
– Automatically generating diagnostic and billing codes eliminates the significant administrative burden typically associated with quality reporting, prior authorization, and other processes that are central to documentation in the value-based care model.
Technology as a tool, not a burden
The healthcare industry has a well-deserved reputation for being reluctant to embrace change. This is not due to an irrational love of the archaic or fear of technology. Quite the contrary. The rapid approval of flexible standards for telehealth use and reimbursement in the early days of the pandemic, coupled with the industry’s fast embrace of virtual care, shows that healthcare can and will pivot when the value proposition and the incentive model for technology adoption are clearly defined.
The same will be true of data aggregation and decision support for organizations that embrace value-based care models. Applying artificial intelligence to clinical workflows behind the scenes can ease the burden of data capture at the point of care while making data usable downstream for decision support and a range of administrative functions.
This provides organizations with a single version of the truth that is preserved and that can be leveraged to improve clinical and financial outcomes, which is critical to success in any value-based care model. More importantly, it ensures that using technology no longer gets in the way of practicing medicine and instead serves as a tool that helps clinical staff make the rights decisions for the right patients at the right time.
About Steven Rube, M.D.
Dr. Steven Rube joined Intelligent Medical Objects (IMO) in 2013 and now serves as the Chief Clinical Officer. With over 15 years of experience practicing family medicine, and previously serving as the Chief Medical Information Officer (CMIO) at a large urban hospital in Chicago, Dr. Steven Rube contributes a frontline user’s perspective to IMO’s executive team.
About Intelligent Medical Objects
Intelligent Medical Objects (IMO) is a healthcare data enablement company. From clinical documentation at the point of care to complex approaches to population health management, IMO ensures clinical data integrity and quality—making patient information fit for purpose across the healthcare ecosystem.