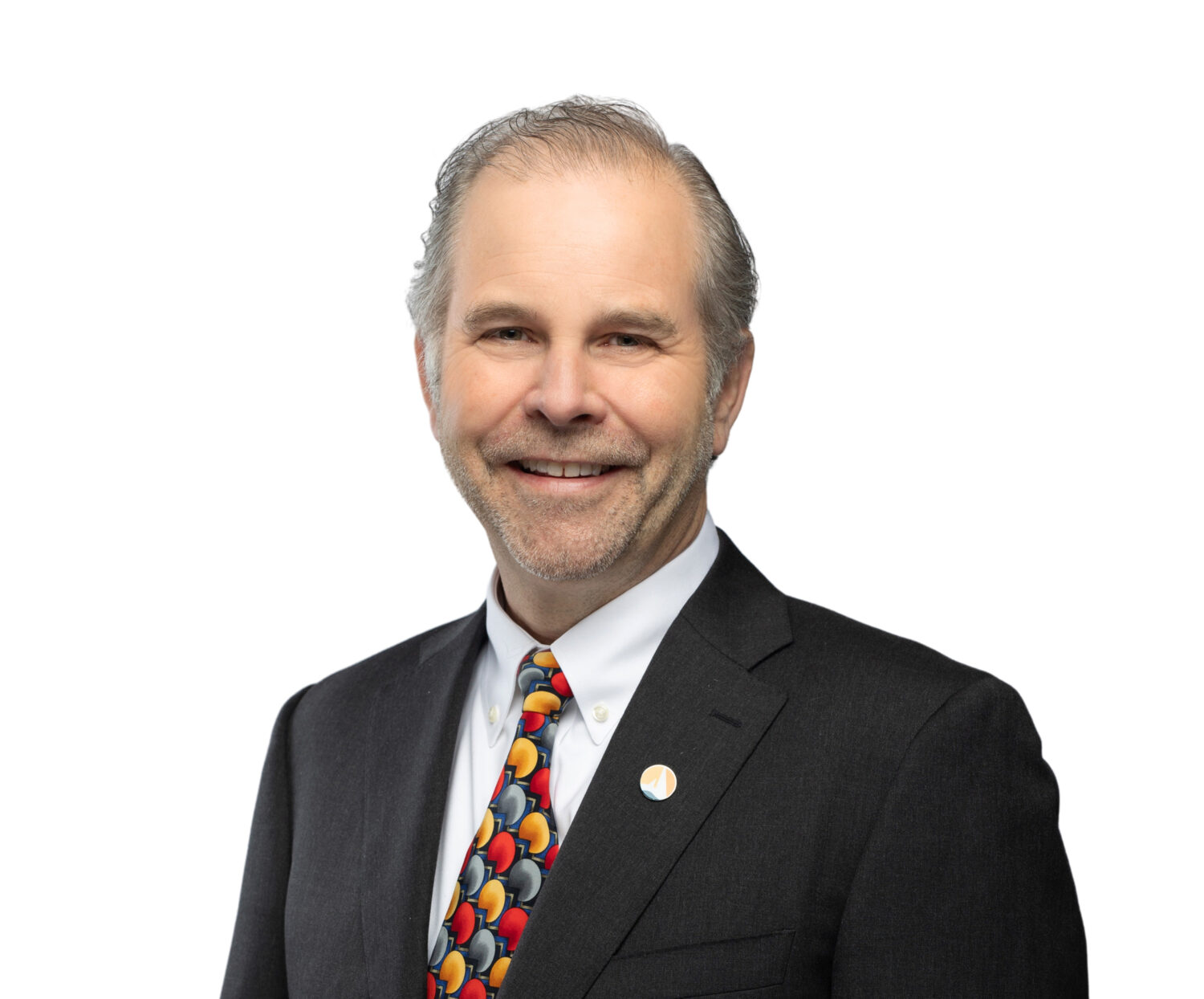
A hot topic we see and hear a lot in healthcare is leveraging big data. Little known fact, we don’t yet have big data in healthcare, so the industry hasn’t had the opportunity to use big data. Healthcare has been in the “little data” game because much of the healthcare experience has yet to be digitized. In addition, interoperability issues leave much data siloed on disparate databases across the healthcare ecosystem.
This is all changing, and this change is accelerating. We’re quickly gaining the ability to un-silo and knit data together, as well as to collect and analyze large amounts of data from patients, providers, payers, labs and other healthcare stakeholders, not to mention digitized medical devices and mobile consumer apps. Data that once was collected at periodic intervals, such as a hospital patient’s pulse, is now streamed continuously to be monitored, stored and analyzed.
We’re collecting more data in an hour than we once captured in the EHR over an entire year. And it’s not just clinical data – it’s coming from nearly every aspect of the healthcare experience. For example, we are getting streamed environmental data such as room temperature, pressure and humidity that is used to automate the monitoring of building health, direct investments in areas with the most significant impact, and provide feedback loops that can adjust the environment to the optimize clinical care experience. This is just one of the dozens of examples of what’s driving healthcare’s data explosion.
The data available to healthcare organizations will increase 100-fold over the next five years.
Given this exponential increase in digital information, it’s clear that healthcare organizations must scale their ability to support that data and break into the big data game if they want to thrive as a destination center.
Most importantly, healthcare organizations need to make this data actionable. By applying analytics to data, healthcare organizations can enable evidence-based clinical decision-making and identify proactive ways to improve outcomes, streamline processes, reduce costs, and accelerate research.
For example, it’s one thing for a hospital to track how many sepsis events occurred in the past week or that day; it’s another approach entirely to anticipate whether a specific patient is going to develop sepsis, based on analytics. Healthcare needs to move decision-making upstream so we can become more predictive and anticipate what’s going to happen before it does, and more prescriptive to guide actions. Data analytics allow us to do just that.
Machine learning also has tremendous potential in healthcare because it can use data to create disease models that help clinicians predict whether patients might eventually develop a specific condition. To do this, however, requires massive, high-quality data sets that most healthcare organizations simply don’t have, yet. But that is changing as the size of data sets grows. The key to success is implementing an operating model and data platform that will allow one to start with small datasets using traditional analytic methods to drive immediate value that will evolve to leverage big data and ML.
3 steps to accelerate your analytics initiatives
Whether a healthcare organization has an established analytics program, is just starting one, or still is in the planning stages, a strategic and systematic approach to data analytics is essential to frame the journey and take full advantage of the digital revolution in healthcare. Here are 3 must-implement steps healthcare organizations can take to accelerate their journey:
1. Frame the journey
Begin the journey with an Action Plan. This critical first step frames the data innovation program. The Action Plan aligns stakeholders on the current state of the environment, vision going forward, and game plan for getting there. It is a living document and evolves to reflect lessons learned. Elements of the Action Plan include business context, current state, program charter and branding, operating model and a twelve-month roadmap.
The effort to create the Action Plan is time-boxed. It is never more than 90 days and is something that can be created by a part-time team member working solo. Most organizations have one or more assessments in place. So, it is best to build off of this to work to accelerate the process. If there are no assessments in play, I recommend a quick assessment using HIMSS Analytics Adoption Model for Analytics Maturity (AMAM) or the International Institute for Analytics (IIA) Analytics Maturity Model. These assessments will provide helpful guidance and can be used to benchmark progress.
The Art of Action by Stephen Bungay provides great insights and examples on how to frame the journey and lead teams that promote adaptability, productivity, innovation and employee satisfaction. A useful complement is Drive by Daniel Pink. This book dives deeper into the power of intrinsic motivation.
2. Build on small wins
After aligning stakeholders on the Action Plan, it is time to work on a pilot. This pilot provides an opportunity to demonstrate value quickly, and to learn and evolve the operating model. This will be the foundation that future efforts will build upon. So, it is crucial not to rush this pilot. It is also essential to be patient. This first implementation is always a little bumpy as people learn a new way of working.
It’s best to conduct your pilot in an area where employees are willing participants. This ensures that the executive sponsors and team members are passionate and committed to the team’s mission and working on something critical that will impact the business – nothing is more motivating. Equally important, the team must demonstrate the visible value that justifies the investment.
Quality and Safety or Health Equity are significant areas to start because they are a high priority in many healthcare organizations. Once your new analytics operating model creates value around the chosen area, a healthcare organization can repurpose or add additional teams to focus on surgical services, business operations, translational research or other areas. Analytics programs can often be expanded without adding many (or even any) resources using data analysts already embedded in the organization.
As the program evolves, a single version of the truth is formed and more and more effort is focused on leveraging this truth to create business value. This is where delivery accelerates. More value is created at a lower cost. This is invaluable when organizations are financially constrained or must navigate challenging economic times.
Agile Analytics by Ken Collier is a very practical reference tool for running agile delivery teams using a product management framework.
3. Power data innovation
Indeed, mature organizations that think strategically often add resources to their analytics programs during difficult economic times because they can use analytics to streamline operations and create automation within workflows. Not only does this commitment to data innovation help organizations survive during difficult economic times, but it also sets them up to ride the wave of economic recovery.
In contrast, less mature organizations will treat analytics as overhead rather than a strategic tool and reduce analytics budgets during or before downturns. As a result, they are poorly positioned to drive efficiencies to survive the economic downturn and to capitalize on the data innovation to thrive during the economic recovery.
In addition to the investment, how an analytics community operates makes all the difference. The nature of the work is data innovation. So, the operating model needs to promote this innovation. This involves a more federated approach where small, loosely coupled teams work in lockstep with the business on areas with the most strategic and operational value. At the same time, these autonomous teams need to leverage and evolve a common data and analytics platform.
More healthcare organizations are looking at this federated, product-driven approach instead of a project-driven one. This is referred to as the digital operating model, and data, analytics and AI are at the center of powering data innovation. In the book Competing on the Age of AI, Marco Ianansiti and Karim R. Lakhani do a wonderful job describing how this operating model has disrupted nearly every industry…but healthcare. In Fail Fast, Learn Faster, Randy Bean does the same for traditional companies…including healthcare.
Under an innovation-driven digital operating model, the goal isn’t to complete a set of tasks at the end of the day. Instead, it’s to create something the organization can leverage to drive value. Everyone is focused on the true customer (i.e., patients and families), business and technology function as one, and decision-making happens where the work is done. In this environment, people aren’t relegated to specialized roles. Instead, they wear multiple hats. A data engineer might be doing data analyst work, or clinicians may be working side-by-side with data scientists, data engineers and operators to build a product that can create value within the organization.
An innovation mindset is needed to successfully implement a digital operating model. While common in the startup world and digital-native organizations, the innovation mindset is gaining a foothold in healthcare as more organizations embrace value-based care and explore new ways to accelerate research.
Conclusion
Powerful forces for change will transform the healthcare landscape for decades to come. This is driven by powerful forces like value-based care, virtual care, consumerism, data interoperability, systems medicine, and emerging technologies (e.g., cloud, IoT and 5G). As these forces mature, massive data volumes are being generated that can be leveraged to dramatically improve the healthcare experience and outcomes, improve provider experience, reduce the cost to deliver care, accelerate research, and promote clinical research integration.
Fragmented analytics efforts lacking strategic cohesion undermine these forces for change, leaving healthcare organizations struggling to respond and survive – and this will only get harder and harder. To capitalize on this opportunity, organizations need to frame their analytics journey practically, deliver business value quickly, and evolve as the organization’s data fluency grows.
To support this Action Plan, organizations need a big data platform that allows them to create a single version of truth, evolve to capture and manage massive datasets, and allow analytics and AI at scale.
To power this transformation, organizations need to adopt a new way of operating that promotes data innovation. This digital operating model leverages a federated approach to governance, an agile, product management approach to delivery, and an intrinsic way of motivating teams.
In thinking about the future of medicine and its potential, Deep Medicine by Eric Topol is a must-read. Eric does an excellent job putting into perspective the power of analytics and AI beyond basic reporting. This is the opportunity ahead. We have the tools and means to deliver. All we need is the will and courage to do it. We must do it. Our family, friends, and communities are counting on us.
About Ryan Sousa
Ryan Sousa is a senior leader with 30+ years of cross-industry success with a passion for partnering with organizations to power data innovation that leverages analytics/AI at scale to streamline operations, promote innovation, accelerate research, and enrich the consumer and employee experience.
His experience includes serving as SVP for two start-ups acquired by Amazon and Nokia. In addition, Ryan led the successful implementation of large-scale analytics at Amazon, Expedia, Starbucks, and MCI. This involved leading strategy, implementation, operations, and commercialization. More recently, he led the analytics transformation at Seattle Children’s as their Chief Data & Analytics Officer, raising the level of maturity from HIMSS AMAM 2 to 7 in four years – the 5th organization to achieve this level of maturity. This included the first commercial software spin-off, AdaptX, where Ryan is co-inventor and board advisor.
Ryan was a faculty member at The Data Warehouse Institute (TDWI), taught Healthcare Analytics at the University of Washington. He’s consulted globally, assisting companies in defining and implementing their analytic and CRM strategies.