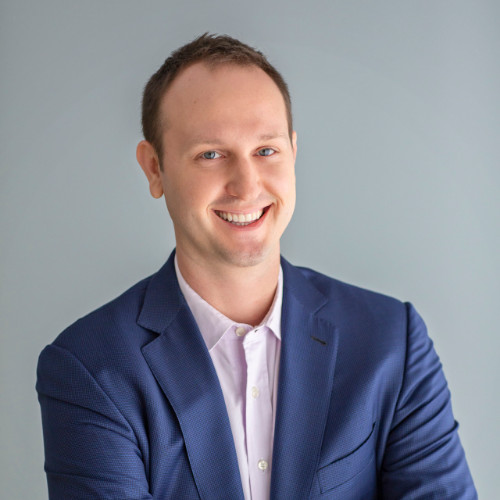
We are entering a new era of patient treatment options thanks to cutting-edge technologies that are changing the way life science companies approach and execute pharmaceutical research. One of the more significant solutions that support the faster and more efficient development of new pharmaceutical products – such as the COVID-19 vaccine, which was developed faster than any other vaccine in history – is artificial intelligence (AI)-driven data analysis. Thanks to modern life science technology solutions that employ AI for data analysis, new treatments for various illnesses can be made safer, faster and more focused on specific conditions.
21st Century Patient Treatment for Rare Illnesses
It can be difficult to develop treatments for patients dealing with rare illnesses, as it is often difficult to find enough patients to conduct thorough clinical research. Further, because the condition is rare, there may be sparse literature on the illness and even fewer specialists to consult. Yet, in many cases, there are more treatment options or clinical research in different stages – the issue is that they are not widely known as the information is not widely shared.
Prior to AI-supported digital technology, the volume of treatments in clinical research environments was difficult for providers to assess as opportunities for their patients. Today, cloud, data and analytics technologies enable healthcare professionals to tailor treatments to a patient’s specific multi-omic data profile. These tailored treatments can be either pre or post-market, which opens up potentially life-saving clinical trials to patients with rare diseases, as the AI-technology supporting data-driven decisions can guide the physician through the huge volumes of patient data and treatment options. Imagine trying to navigate the internet without the support AI search algorithms and you can understand the challenge facing a physician trying to navigate the modern clinical research options at the same time as their patient’s multi-omic data profile.
Making Safe Data-Driven Decisions with AI
While AI-powered data analysis makes clinical research more efficient, it does not sacrifice safety for speed. Although manual efforts to analyze clinical research information are reduced, a large amount of data must be collected for accurate algorithm development. Any fragmented or inaccurate data diminishes the positive impact of AI-supported clinical research and could potentially result in unsafe data-driven decisions.
The key to making these patient treatment decisions is analyzing enough diverse data to gain insights into safety signals and the efficacy of new drugs. Disparate data from various sources will inevitably need to be cleaned and standardized for analysis. AI helps to support this process of data collection and preparation by automating the compilation, standardization, and analysis of all the data. This automatically establishes a universal source of truth, without the risk of human error to offset the accuracy of machine-generated insights, for healthcare professionals, regulators, and clinical researchers.
This data synthesis helps to overcome a significant challenge for today’s clinical researchers, who must compile reports from HCPs and digital data from labs to get the full picture of a medicine’s effectiveness and safety. In addition, different countries have different regulatory requirements for handling patient data. For example, European servers must comply with GDPR guidelines, and in China patient data cannot cross the border to another country. Leveraging AI to automate the collection of the appropriate data can ensure that the right information is collected and analyzed to meet regional requirements and ensure patient safety.
Making Clinical Trials Data More Accessible for HCPs & Patients
Clinical researchers and pharmaceutical organizations tend to use digital technology to record and store data, the systems that HCPs use are rarely connected to those used by pharmaceutical organizations. In fact, there are dozens of different computer systems that clinical researchers and physicians use to record information. This situation creates siloed data on both ends of the spectrum. HCPs cannot easily access clinical research information, and researchers cannot easily receive updates on the efficacy of their new treatments from HCPs.
Currently, patients can also seek clinical trials that may offer novel treatments and therapeutic combinations intended to reduce symptoms and the overall burden of disease by visiting ClinicalTrials.gov. However, the website is not particularly patient-friendly and doesn’t make finding relevant and potentially effective trials easy for patients that are not medically trained or educated. It is much easier for HCPs to identify clinical trials for their patients, especially when they have access to portable, AI-based technology at their fingertips that could suggest clinical trials for their patient’s respective illnesses.
Cloud and AI technology allow life science companies and HCPs to collaborate, creating a seamless map of a patient’s journey through the treatment process to further future research and develop newer and more innovative treatments for rare diseases.
A Better Future for Rare Disease Patients
Today HCPs and clinical research organizations weave together patient and treatment data while leveraging AI analytics, to safely place patients in clinical trials, as well as speed the development and approval of new medicines. This technology allows healthcare professionals to spend more time treating patients, and less time diagnosing their illness and researching a possible treatment. Companies that adopt AI-driven analysis for clinical research will also be better positioned to develop newer and more effective treatments down the line.
About Luca Glass
Lucas Glass is the Vice President of the Analytics Center of Excellence at IQVIA. The Center is responsible for researching, developing and operationalizing machine learning and data science solutions in the R&D business. Lucas started his career in pharmaceutical data science 15 years ago at Center (then owned by Galt Associates), working on pharmacovigilance data mining algorithms. Since then he has worked at the U.S. Department of Justice in healthcare fraud, several small startups, and TTC, LLC, which was acquired by IMS Health in 2012.