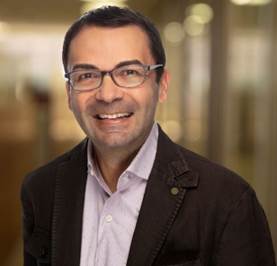
Artificial intelligence (AI) is making a tangible difference in healthcare today. It’s not about science fiction or flashy gimmicks. It’s not about deep fakes or plagiarized term papers. AI is being responsibly used to prevent medical errors, enhance clinical decision-making, expand access to care, and lower costs. While there’s certainly overenthusiasm and misleading claims about AI, we can’t ignore the many instances where it’s making healthcare more efficient, effective, and patient-centered.
AI is Not New, But Its Impact Is Accelerating
The roots of AI go back centuries, with the first predictive algorithm credited to the German mathematician Carl Friedrich Gauss in 1795. However, it’s only in the past decade that AI and machine learning (ML) have truly taken off, thanks to exponential advances in computing power and data availability. The true potential (and risk) of AI and ML algorithms have accelerated with a number of unique applications and approaches being developed. Not all AI is the same and can simply be classified into predictive, prescriptive, and generative AI/ML, with the latter creating the most excitement and controversy over the last 12-24 months. Today, these technologies are being used to predict rising health risks, recommend treatment options, and even generate new medical insights.
PCCI: Leading the Way in Responsible AI in Medicine for Underserved Populations
At PCCI, we’ve been researching and testing AI in healthcare for over a decade, with a focus on serving the most vulnerable populations. Our approach is rigorous and scientific, ensuring that clinicians are always in control, decisions are transparent, and patients are at the heart of everything we do. We believe that AI can truly transform healthcare, but only if it’s developed and used responsibly.
PCCI has created a healthcare-focused, secure, and private digital platform called Isthmus™, where healthcare data can be safely stored and analyzed using cloud technology and industry-standard tools. The platform is deployed behind an institution’s firewall to ensure that no PHI data is ever exposed to the outside world. This protected environment ensures the confidentiality and security of sensitive patient information while enabling advanced analysis and modeling capabilities.
When building AI/ML models, PCCI relies on a core set of principles:
- Clearly articulate the problem: Ensure the AI is solving a real problem and not just engaging in “cool math.”
- Assemble a multi-disciplinary team: Include from the start a passionate lead clinician, operational experts, technology specialists, and legal/compliance reviewers.
- Prioritize data quality and relevance: Curate, validate, and analyze diverse data that accurately reflect the patient population.
- Leverage a secure data environment: Utilize a dedicated and reliable digital sandbox environment (like PCCI’s Isthmus), separate but linked to the Electronic Health Records (EHR).
We understand that accuracy is paramount in healthcare, which is why we take a careful and methodical approach to both developing, deploying, and monitoring healthcare applications. Our processes prioritize patient safety and reliable results:
- Building Models: We create models using historical data that is representative of the specific patient population, ensuring that the model is tailored to the unique needs and characteristics of the people being served.
- Testing and Optimizing: We rigorously test each model with a separate “hold back” set of data, refining its performance based on valuable feedback from clinicians. This step ensures that the model not only works in theory but also functions effectively in the real world of clinical practice.
- Phased Deployment: We deploy a model with live data but run it in silent mode before exposing it to clinicians. No decisions are made using the model, but the model’s performance, stability, and expected output is evaluated and monitored. We also evaluate the model for equity and expected performance on the respective patient population. If the model is built with a different data set, we ensure that it performs as expected in the specific patient population of interest or we go back and re-train the model. This could take months or longer. When you are trying to predict a rare event, it could take years to ensure an adequate amount of data has been captured to build a reliable model. For example, to ensure proper evaluation for the PCCI Parkland Trauma Index of Mortality (PTIM) model, we went into a full silent mode on every patient, every hour, for more than 6 months before we moved to provider-facing production.
- Deployment and Monitoring: Once the model demonstrates its effectiveness in silent mode, it’s time to deploy it into the clinical workflow. However, work doesn’t stop there. We continuously monitor model performance, evaluating its impact on the patient population, and making necessary adjustments to ensure it delivers the intended benefits over time.
- Integration and Transparency: PCCI developed Islet™, a web-based, rich, model visualization tool that seamlessly integrates model results into existing systems, such as EHR or case-management systems, making it easy for clinicians to access and utilize the insights generated by the model. It requires no additional logins or workflow changes. We believe that models shouldn’t be “Black Boxes” and Islet was developed to allow us to prioritize transparency by providing clear explanations of the important actionable factors that influence a model’s predictions.
- Gradual Implementation: We understand the importance of a smooth transition. Therefore, we adopt a phased approach to implementing the model, starting with education and training of specific teams or departments, and gradually expanding its use. This allows for continuous evaluation and feedback to ensure successful integration into clinical practice.
- Unplanned Model Downtime Process: The power of AI is tangible and useful and clinical teams come to rely on AI/ML model assistance. It’s like getting used to navigating using your car’s integrated GPS system and then having to go back to using a map. You can still drive and get to your destination, but it’s not as easy. Make sure to implement a process to address off-cycle downtime such as regular updates and maintenance or unexpected system disruptions. Depending on how the model is used and how often the data is refreshed, model-specific service level agreements (SLAs) need to be created to ensure rapid response and coordination between the technology, operational, and analytics/modeling teams. Clinical decision support models that have 15-, 30-, or 60-minute data refresh rates, such as sepsis risk predictions, require very rapid SLAs within hours.
- Ongoing Maintenance: Model success doesn’t end with implementation. Deploying a model is not a “one-and-done.” It requires ongoing support to regularly evaluate, test, and update the model to ensure it remains accurate and effective over time, adapting to the evolving data and the needs of specific patient populations.
It’s crucial to re-emphasize that AI and ML are tools designed to augment, not replace, the expertise and judgment of healthcare professionals. Our mission is to empower healthcare teams with the information and insights they need to make informed decisions and deliver the best possible outcomes and care to their patients.
Key Takeaways for Healthcare Leaders
- AI is already improving healthcare: AI is being used to prevent harm, enhance decision-making, expand access, and reduce costs.
- Responsible AI is essential: AI should be developed and deployed with transparency, clinician oversight, and patient focus.
- Look beyond the hype: While there’s excitement and some overblown claims, focus on the real-world impact AI is having in healthcare.
- AI is a tool, not a replacement: AI should be used to augment, not replace, the expertise and judgment of healthcare professionals.
- Model deployment is as important as model development: While powerful tools like Isthmus™ and Islet™ are great for building AI/ML models, the best model in the world is useless if it can’t be effectively deployed and integrated into a clinician’s workflow.
Whether everyone knows it, understands it or even likes it, AI is here to stay. It is exploding in healthcare and increasingly making a huge difference in our lives. At PCCI we will continue to focus on applying and localizing these powerful concepts with those who serve the most vulnerable individuals and communities. That’s our mission and focus and will remain that way. We also cannot and should not do it alone. There are many leading innovators and pioneers across the country building, testing, and evaluating new applications and developing the right guardrails for responsible, ethical, and equitable applications of AI. The Health AI Partnership is one of the leading coalitions of AI innovators focusing on collaboration and knowledge sharing to empower healthcare professionals to use AI effectively, safely, and equitably through community-informed, up-to-date standards.
Agreat collection of curated, best-practice guideson AI life cycle management that are generally applicable and broadly vetted can be found at Health AI Partnership (HAIP) (Health AI Partnership Publishes Best-Practice Guides | Healthcare Innovation).This is a constantly growing portfolio of information and should be accessed early and often. A few of my current favorite pieces are:
- A guide for AI Implementation: 8 decision points to consider when implementing an AI solution.
- A framework for mitigating the risk of AI solutions worsening health inequities: Development and preliminary testing of Health Equity Across the AI Lifecycle (HEAAL).
- A review of the FDA’s guiding principle for Good Machine Learning Practices (GMLP) for developing and deploying ML models.
About Steve Miff
Steve Miff is the President and CEO of Parkland Center for Clinical Innovation (PCCI), a leading, non-profit, artificial intelligence and cognitive computing organization affiliated with Parkland Health, one of the country’s largest and most progressive safety-net hospitals. Spurred by his passion to use next-generation analytics and technology to help serve the most vulnerable and underserved residents, Dr. Miff and his team focus on building scalable solutions for responsible applications of AI in Medical Care for underserved populations. He was the recipient of the 2020 Dallas Business Journal Most Inspiring Leader award and the winner of the 2021 DCEO and Dallas Innovates healthcare awards. Dr. Miff was also named to the 2020-2023 Dallas 500 Most Influential Leaders Awards. In 2023, he was named the Tech Titans emerging company CEO of the year. Under his leadership, PCCI was named one of the 2019 Dallas Best Tech Startups by the Tech Tribune, the award recipient for the 2022 Corporate Citizenship Award, and through Parkland Health, received funding from the prestigious Augmented Intelligence in Medicine and Healthcare Initiative award by the Kaiser Permanente Division of Research.
In addition to local leadership, Dr. Miff is playing an influential role with C-suite leaders across the country. With the emergence of AI innovation in healthcare, he has and is continuing to play a major role nationally for the responsible, ethical, and equitable applications of AI. Dr. Miff is an active member on the National Academy of Medicine AI Adoption and Code of Conduct Committee, Advisory Board Member for the Health AI Partnership in collaboration with Duke, Mayo, UC Berkeley and DLA Piper, a Senior Fellow on the Health Evolution AI Collaborative, and serves on expert panels and listening sessions for NIST and White House AI policy initiatives.
*(Contributors to this article include Russell “Rusty” Lewis, Executive in Residence at PCCI, and Albert Karam, PCCI’s Vice President, Data Strategy and Analytics.)