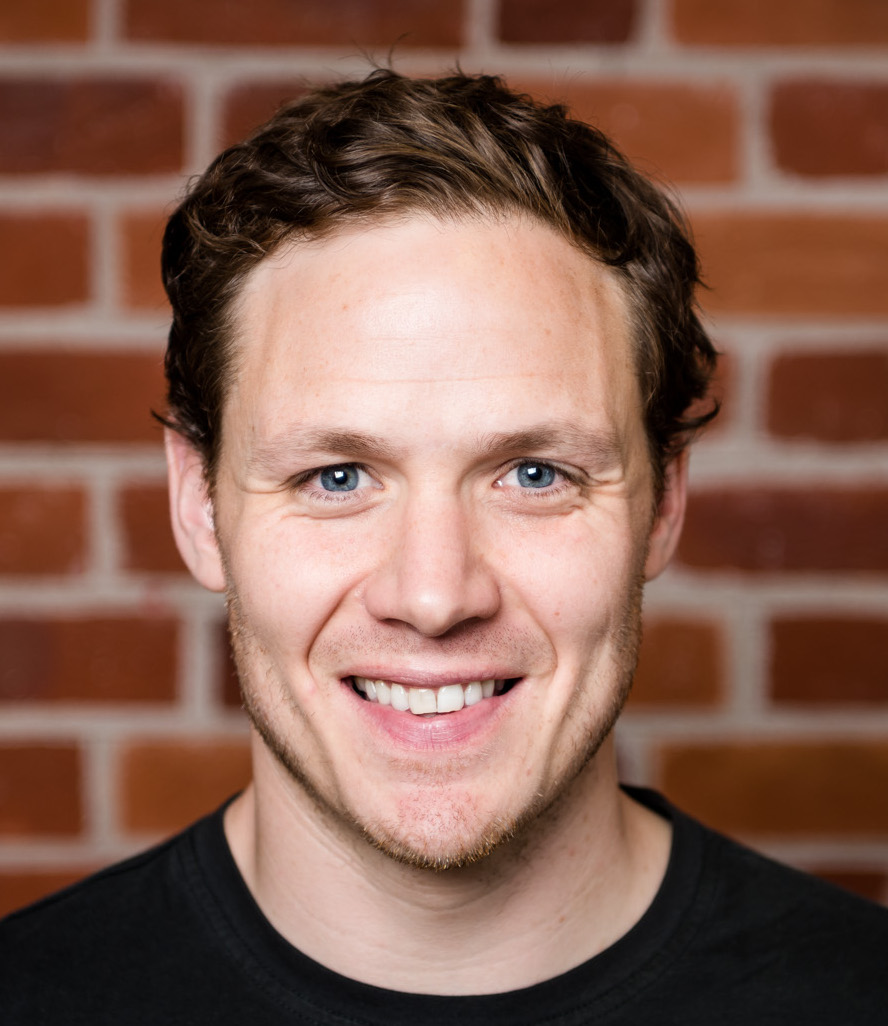
The promise of personalized medicine is a world in which interventions, screening, and therapeutics are targeted to those most likely to get the disease. Polygenic risk scores will help society reach this ambition by accurately assessing the contribution that one’s DNA makes to disease risk.
When Thomas Jefferson wrote in the Declaration of Independence that ‘all men are created equal,’ he captured the essential concept of equality on which the US Constitution is based. Although no one would argue with the importance of this idea, when it comes to our health, it doesn’t exactly hold. Some of us will live long lives in relatively good health, while others will succumb to the disease. So, when it comes to one’s susceptibility to disease, unfortunately, we were not all created equal.
Thanks to decades of medical research, we now understand that disease is not a completely random process. People who smoke are more likely to get lung cancer. A poor lifestyle increases your risk of many common diseases. And some diseases run in families — meaning that knowing the diseases of your relatives can help you understand your chances of getting the same disease.
Many factors affect your risk of disease. These include aspects of your environment and behavior that are within your control, and others that are fixed, such as your genetics. Since the human genome was sequenced over 20 years ago, enormous strides have been made in understanding how variation in the DNA code within us affects our chances of disease. This has led to a step-change in the way in which this source of data can be used to help identify those at greatest risk of disease.
Take breast cancer as an example. If you carry a mutation in one of the two BRCA genes that were discovered in the 1990s, you are at 5 to 10 times increased risk of breast cancer compared to a non-carrier. Such is the effect of mutations in these genes, that testing for the presence of these mutations is now routinely performed in eligible women with a family history of breast cancer. However, across a population, these mutations are incredibly rare and are carried by roughly 1 in 200 people.
It is now possible to construct a polygenic risk score (PRS) to identify how genetic variation across the genome affects your chances of developing breast cancer. Rather than focusing on rare pathogenic mutations, PRSs capture the effect of hundreds to millions of common genetic variants across the genome. Each of these variants may have a small effect, but when aggregated into a single score represent a powerful approach for assessing the genetic component of disease risk.
A PRS for breast cancer can identify significant proportions of the population at heightened risk of disease. For example, polygenic risk scoring can be used to identify around 1 in 5 women who are at twice the risk of breast cancer as an average woman. As a point of reference, family history is a well-established risk factor for breast cancer that also increases relative risk by two times in the case of one first-degree relative with breast cancer.
We now have the power to assess someone’s DNA and to understand their risk of breast cancer, but what needs to be done to bring this type of test into routine clinical practice? The three most important and pressing issues are: understanding the ethnic biases in PRSs and ensuring that they are accounted for, developing appropriate guidelines for the implementation of PRS in primary prevention, and aligning best practices for the reporting of PRSs to patients and clinicians.
Ancestry Bias
Across medicine, there are examples of clinical models whose performance varies in different populations. The commonly used cardiovascular disease 10-year risk estimator, the Pooled Cohort Equation, for example, is known to be less accurate in individuals with South Asian or African American ancestry. Ancestry bias is pervasive across medicine and models built with genetic data are no different.
Most genetic data generated to date come from individuals of European ancestry. This poses a challenge when developing tests that link variation in genetics with disease risk for two main reasons. The first is that when we find associations between DNA and disease, the particular variant identified as having an effect on disease is often not the actual disease-causing variant. Instead, it is located close to the causal variant in the genome. Because current global populations are the result of human migrations over the last 200,000 years, some groups have spent long periods of time apart. This time has allowed DNA to diverge across populations, such that the variants that are close to causal variants can be different depending on your ancestry. The result of this is that those variants that tag causal variants in one population don’t always tag them in another.
The second reason is that the frequency of both tagging and causal variants differs in different populations, so individuals can have more disease-associated variants not because of increased risk of disease but because they happen to have more of the variants.
What this means is that we need to proceed with caution when applying models developed in one population to another. What it doesn’t mean is that we should not use such models. As datasets grow and methods are further developed to bridge the gap, there is every hope that models will perform better across all populations. In the meantime, we can validate PRSs based on different populations to objectively test how well such models work across ancestries. Typically, in situations where PRSs have different performances in different ancestries, we can still identify individuals at increased risk, but the proportion of such individuals might be smaller.
Clinical Guidelines
PRSs have been developed for hundreds of different traits and diseases. However, in most cases, the evidence for clinical utility is limited at best. Nevertheless, there are several examples of PRSs for common diseases where the evidence for their clinical utility is stacking up. In fact, in a few cases, including breast cancer, prostate cancer, and coronary artery disease, this evidence is now sufficient that the argument is not if but when polygenic risk scoring should be incorporated into primary prevention.
PRSs for these diseases, as well as few others, have similar properties: they can strongly stratify risk across ancestries, there is already some genetic testing conducted on them, and there are clear ways in which the outputs of PRS-integrated risk assessments can be used. These include increased or earlier mammographic screening for breast cancer, increased surveillance for prostate cancer, and interventions that target modifiable risk factors for cardiovascular disease. Importantly, there are also already clear national guidelines on what to do if you are at high risk. So PRS can be used to augment clinical pathways that already exist by adding precision to current risk assessments.
Reporting Standards
When it comes to reporting PRSs, there are several options. Understanding the increase in your relative risk of disease—for example knowing that your genes increase your risk by 2, 3 or 4 times the average is a common way in which the risk from non-genetic risk factors is communicated, so PRS-based recommendations can align with what patients and clinicians are already familiar with.
Thanks to the availability of large, diverse, and detailed datasets, it is also possible to build and validate absolute risk models of disease. These can include estimates of 5-year, 10-year, and lifetime risk of disease, and may or may not also incorporate additional known risk factors. The outputs of such risk assessments can provide individuals with an understanding of their risk of disease.
Ultimately, PRSs are a tool for capturing an increasingly accurate assessment of your genetic risk of disease. When combined with other risk factors, these can form a potent mechanism for identifying anyone’s risk of several common diseases. By identifying those at higher risk, on whom interventions can be targeted, PRSs are central to the ambitions of precision medicine. As such, they will also be key to reducing the burden of common diseases, allowing us all to lead longer, healthier lives.
About Dr. George Busby
Dr. George Busby is co-founder and chief scientific officer at Allelica, a company that builds genomic analysis software for health systems and genetic labs. After postgraduate studies at Imperial College London and doctoral research at Oxford University, he spent over ten years studying the links between the human genome, ancestry and disease risk in Oxford. He is passionate about translating the enormous advances in human genomics into tools that can reduce the burden of preventable common disease. Contact George at george@allelica.com.