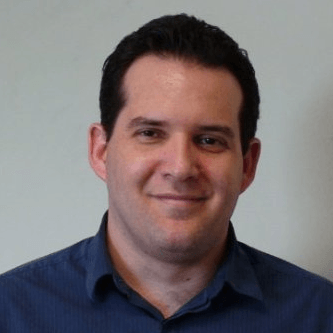
Heart disease is the leading cause of death for people of most racial and ethnic groups in the United States. Cardiovascular disease-related deaths—which occur every 36 seconds—cost our country about $219 billion each year, according to the Centers for Disease Control and Prevention (CDC). People with poor cardiovascular health are also at increased risk of severe illness from COVID-19, so the time to act is now. There’s no time like the present to look at major risk factors—from obesity and smoking to high cholesterol and blood pressure—and how to avoid them.
While acute care and medications exist to treat heart disease and other cardiovascular conditions, too often we look at how to manage ailments that already exist, rather than how to prevent them in the first place. While heart disease does affect a massive group of the population, like many diseases, it does discriminate, and without looking at the full spectrum of a patient’s life, it’s impossible to get to the root cause. In recent years, natural language processing (NLP) technology has been used to analyze social determinants of health to uncover helpfully, or potentially dangerous, information about patients that may help us understand more about the disease.
Related: 8 Use Cases for Natural Language Processing (NLP) Technology in Healthcare
Social determinants are elements that directly impact a person’s health beyond diseases or drugs, such as access to healthy food, personal safety, housing, employment, literacy, family, employment, and personal freedom. These are often more important than clinical treatment when it comes to managing chronic diseases, like heart disease, and a slew of other medical conditions. The challenge here is that social determinants can often only be read from free-text notes in a healthcare setting—not in structured data. In order for medical professionals to realistically compile and use this information, they need NLP.
Here’s why: doctors aren’t social workers, and in most cases, there’s no structured way to ask about social determinants. Without structured data, a lot of the pertinent information about social determinants will be in patient notes. Doctors will manually write about a patient’s social history, home environment, and similar types of health contributors. Structured data in electronic medical records (EMRs) would only consist of lab results, billing codes, and what medications the patient is taking. But if there’s substance abuse, unemployment, homelessness, or illiteracy, those will be in the notes. NLP is the automated way to connect the tissue between these disparate and siloed data sources to understand how these health events are related.
In addition to the challenges of connecting free-text and structured data, sometimes, medical professionals simply don’t know what they’re looking for. Let’s say you want to do longer-form studies about what happens to patients with heart disease. Do their symptoms improve if they take vitamins and exercise regularly? They may—and if that’s what you’re looking to prove, that’s great. But NLP is the only viable way to correlate all potential variables—sleep, relationships, safety, employment, obesity, etc.—to get real answers. It would be impractically time-consuming to read line-by-line and try to connect the dots, even if all the information you needed was in the text. But what if you need to consider diagnostic imaging reports, or social media behavior, too? You need software to contract the relationship between these things.
There are also questions about the quality of data. Fortunately, cardiology is well-known for using data-centric governance models. The American College of Cardiology cardiac catheterization and angioplasty initiated its data registry in 1994. That was a preliminary step after which the CathPCI registry of the National Cardiovascular Data Registry (NCDR) started its duties 25 years later, taking the charge of 90% of cardiac-related data in the US. This regulatory body governs the quality enhancement process with regard to the procedures and outcomes in many healthcare organizations. Quality data is critical for providing accurate analytics.
Despite this, data integration is still a problem in large research projects where information is collected from different entry points and data is available in different formats, and some are missing, or inaccurate. Once again, NLP is an excellent source for researchers working in the cardiology field to mitigate this issue. With existing datasets in this specialty, researchers and data scientists can more easily glean insights or uncover new findings with increased accuracy. Having curated and standardized data can make researchers’ jobs much easier and save years of headaches.
Social determinants are a huge part of public health and are often undercounted when exploring chronic illnesses, like heart disease. A woman who is dealing with domestic abuse at home isn’t going to be prioritizing her diet and exercise regimen to manage her heart health. A man who is unemployed and lost his health insurance may start missing important follow-up appointments in order to defray costs. Being aware of these social indicators and using them to inform care—whether prevention or management of heart disease and other illnesses—is vital for patients’ overall health outcomes. Technology like NLP has made it easier to start correlating social determinants to heart health and has the potential to vastly improve prevention and treatment if applied correctly and ethically.
About David Talby
David Talby, Ph.D., MBA, is the CTO of John Snow Labs. He has spent his career making AI, big data, and data science solve real-world problems in healthcare, life science, and related fields. John Snow Labs is an award-winning AI and NLP company, accelerating progress in data science by providing state-of-the-art models, data, and platforms. Founded in 2015, it helps healthcare and life science companies build, deploy, and operate AI products and services.