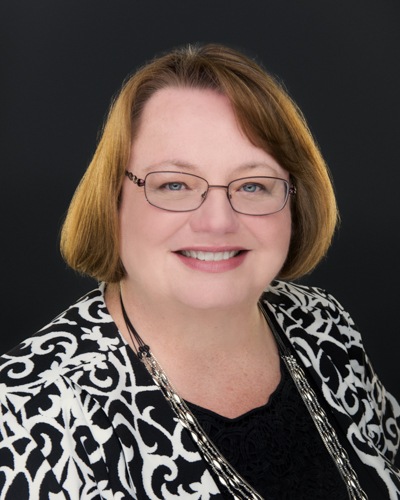
Clean patient data is the critical first step for any successful health IT integration, EHR conversion, interoperability initiative or electronic information exchange. According to a recent Pew Charitable Trusts report, most hospital executives and administrators believe the accuracy of patient matching when information is exchanged between organizations with different EHR systems is subpar.
Further, as health systems continue to consolidate and add more data to their networks, the importance of maintaining accurate patient data continues to grow. Mismatched medical records can lead to fragmented care, patient and physician dissatisfaction, and billing errors. Correct, complete records are essential for proper patient diagnosis and treatment as well as hospital reimbursement and HIPAA compliance.
Prevention of patient identity issues during EHR consolidations requires early detection of duplicate and overlapping medical records. Many organizations rely on enterprise master patient index (EMPI) software and cleanup services to link patient data across multiple systems. Today’s sophisticated technologies use probabilistic algorithms to determine the probability that two records represent the same person.
A 2018 Black Book™ poll of enterprise master patient index users revealed that prior to administering an EMPI tool, an average 18 percent of an organization’s patient records are found to be duplicates. And, hospitals with EMPI support tools in place since 2016 reported consistently correct patient identification at an overall average 93 percent of registrations and 85 percent of externally shared records among non-networked providers.
Though this approach has proved effective over the years, patient matching issues have become more complex in an era of provider consolidation, interoperability and health information exchange. Patient misidentification has significant implications for healthcare organizations, including duplicate records, medical errors and reduced revenue. Based on recent research, here are three key areas of concern for healthcare leaders and providers.
1. Patient safety/care
A Ponemon Institute survey, the “2016 National Patient Misidentification Report,” revealed that 86 percent of respondents—including nurses, physicians and IT practitioners—had witnessed or known of a medical error that was the result of patient misidentification. And, 84 percent strongly agreed that misidentifying a patient can lead to medical errors or adverse events.
2. Revenue for the organization
According to a 2018 Black Book™ Consumer Satisfaction Survey, 33 percent of all denied claims result from inaccurate patient identification or information, costing the average hospital $1.5 million in 2017 and the US healthcare system more than $6 billion annually. Further, the average expense of repeated medical care due to duplicate records costs a reported average of $1950 per patient per inpatient stay and more than $800 per ED visit.
3. Patient satisfaction
Mismatched medical records often result in duplicate tests, unnecessary treatment, and privacy and security issues. The consumer satisfaction survey showed that 88 percent of respondents blamed the hospital system directly for the lack of patient record portability and access among providers.
Referential Matching and Why It Matters
Healthcare organizations are now turning to referential matching to expedite the resolution of unmatched patient identity or duplicate records to unique identities. Proprietary linking technology and vast referential data, including records from disparate public and proprietary data sources, can match records to unique individuals to automate services.
Referential matching is a distinct approach that augments the patient matching of EHR and EMPI technologies. It is not a replacement for an EMPI to ensure accurate patient identification. It is fundamentally different. Instead of comparing only the demographic data within the hospital records— which is not updated unless the patient returns—referential matching technology matches patient demographic data to a comprehensive, constantly updated reference database of identities.
Referential data supports EMPI and EHR matching logic by augmenting the healthcare facility data with big data including thousands of sources of public records and proprietary data—such as utility records, phone directories, credit records, data from government and law enforcement agencies—to build a true “golden” record for an individual.
A Combined Approach to Prevent Patient Identity Issues
Healthcare executives and experts who contributed to the Pew report acknowledged that while no single solution is likely to solve the misidentification problem entirely, “concurrent progress on several fronts could begin to address matching deficiencies.” In our experience, the most effective approach includes three essential pieces of the patient matching puzzle—referential data combined with EMPI software and MPI cleanup services to prevent patient identity issues. Data integrity is the highest priority.
About Letha Stewart
Letha Stewart, MA, RHIA is a health information management professional with more than 30 years of experience in the healthcare field including HIM, Emergency, and Radiology. She is currently the Director of Customer Relations for QuadraMed’s EMPI software and services. During her twenty-year tenure with QuadraMed, Letha has performed and managed both software implementation and cleanup engagements and has extensive hands-on experience with EMPI projects of every size and complexity. Letha has worked with AHIMA to develop lessons on the QuadraMed EMPI software tools for the AHIMA Virtual Lab and she has served on the AHIMA Practice Council on Health Information Exchange. She also speaks frequently on the importance of the EMPI in healthcare.