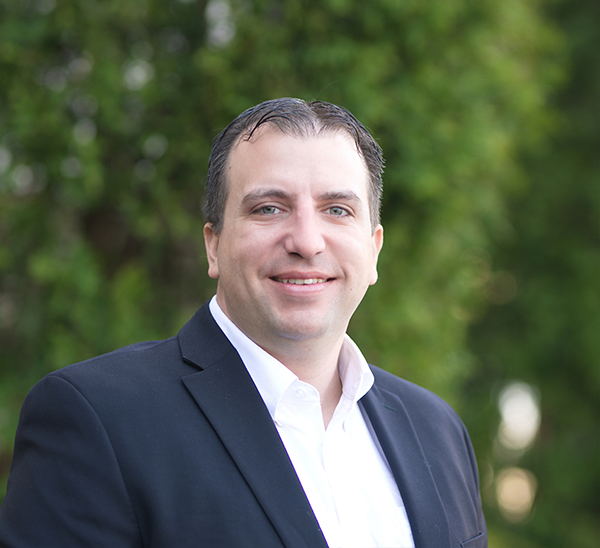
The emerging technologies hype cycle had multiple AI applications at the peak of inflated expectations in 2023 and were just beginning the ride down to the Trough of Disillusionment in 20241 2. For years, it’s been said that “the use of data and algorithms is going to transform healthcare.” While true to a degree, we see that MedTech companies that invest in trends like artificial neural networks, analytics, big data analytics, and now AI, often struggle to yield a significant return on investment. These technologies are undoubtedly valuable, but why do so many organizations fail to capitalize? R&D dollars are precious and represent the fuel for your organization’s future growth.
AI without a clear business model and an understanding of the implementation and management costs is likely to be an expensive program that fails to live up to expectations. Assessing the full impact of any new technology is an imprecise exercise, and delaying participation in the next “gold rush” could leave your business lagging behind competitors. However, spending potentially millions of dollars without a return is a larger risk.
A few common mistake patterns include:
- New Technology Indulgence – Attempting to apply a new technology in too many places, missing a focused strategy.
- Guess and Launch – Informally evolving a proof of concept into a formal product that’s challenging to control.
- Lacking Data Management – In AI, data has a lifecycle, just like software code. The infrastructure to support it is required to be efficient and compliant.
- No Financial Model – Not having a defined connection between your AI initiative and your P&L.
Below are some key steps to help maximize the impact of limited R&D funds, seize market opportunities, and maintain organizational focus, ultimately increasing the chances of AI successfully transforming your business.
Focus on a Problem
Begin with the unresolved problems your customers already face—many of which you likely know. Which of these challenges could lead to cost or time savings for them? In healthcare, AI is often most effective in accelerating decision-making or identifying opportunities to eliminate unnecessary steps.
Define Success
Most of the time success means a positive impact on the P&L, but are you going to get there with a higher average selling price, increased market share, or is there an option to lower your costs?
Healthcare reimbursement is tightly regulated, meaning clinicians are unlikely to pay for a solution unless it delivers time savings, increases patient volume (e.g., more procedures or new indications), or significantly improves outcomes. Prioritizing problems that meet these criteria will enhance the chances of commercial success.
Prove It
AI thrives on data, so a product that lacks connectivity or the ability to generate meaningful data is unlikely to be a strong fit for data-driven AI. Before investing in AI, ensure your product has the necessary foundation.
If the right data sources and connectivity are in place, it’s time to experiment. At this stage, infrastructure investment is minimal, allowing you to use data to validate whether the problem can be effectively solved. You may discover that key data signals are missing or that a critical data point is absent.
Evaluating your organization’s AI capabilities can be challenging. As with any emerging technology, speed is crucial—bringing in external expertise can help accelerate learning and decision-making.
Full Speed Ahead
Reaching this stage means you have identified a strong business opportunity, a clear fit within your product portfolio, and evidence that AI can solve the targeted problem. However, it’s crucial to distinguish experimentation from formal development, especially in healthcare, where maintaining control over development efforts is essential.
AI infrastructure can be as complex as the product itself, requiring well-defined data use cases that support developers, data scientists, and compliance needs, such as privacy. For instance, if a patient exercises their right to be forgotten, how will you remove their data from a series of models? Overlooking these considerations can lead to costly and complex mitigation efforts down the line.
The processes supporting AI will be just as intricate as traditional software development. Version control, provenance tracking, and data deidentification must all be structured, controlled, and repeatable.
AI has the potential to transform not only healthcare but also the way we work every day. However, for MedTech organizations to fully participate in this transformation, they must remain laser-focused on opportunities that deliver precise and measurable value.
About Adam Hesse
A seasoned technical manager and entrepreneur, Adam Hesse brings more than 15 years of Medical Device and Healthcare Information systems experience to Full Spectrum, coming most recently from a leadership role in BD’s Diabetes division, and leading a major modernization program of Medtronic’s CareLink platform before that. Adam has direct and deep development experience in both these industries as well as robotics and automation systems.
References