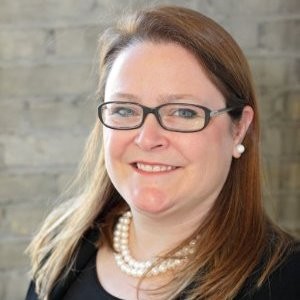
With healthcare data breaches on the rise and concerns about patient privacy escalating, the role of AI in anomaly detection is becoming increasingly critical. To address these challenges and improve patient data security, AI-driven anomaly detection is emerging as a critical solution. To gain deeper insights into this evolving landscape, we recently sat down with Sarah Danielson, Sr. Director of Healthcare Strategy and Advisory at Trace3 to discuss how AI-driven anomaly detection can help protect patient data and improve accuracy.
How do traditional anomaly detection methods fail to keep up with the volume and complexity of healthcare data and what impact do false positives have on the healthcare industry?
Sarah Danielson, Sr Director, Strategy & Advisory, Health Solutions Group at Trace3: Healthcare Organizations today produce high volumes of information, both patient-attributable information, as well as other data types. The explosion of IOT and IoMT in critical infrastructure sectors such as healthcare can bring great benefits, but also new risks, as the proliferation of devices and automation (including Operations Technology and Industrial Control Systems) expands the volume and variety of cyber asset attack surfaces.
Device-attributable information is an especially keen area of interest for advancing IT operations excellence and can include information that can be attributed to the health of a patient, but also, that can be attributed to the device—data about device workloads, device performance, device vendor/ manufacturer, device patching status, device end-of-life date, device location/tracking/placement, device utilization, sector-specific device alerts/notifications, and so on.
Effectively monitoring these cyber asset attack surfaces requires innovative solutions, including AL/ML analytical capabilities. False positives can create unnecessary follow-ups for the security operations center resources, and the legitimate operational users they are investigating.
How can AI and machine learning techniques address inaccurate patient records, and in what ways does AI-driven anomaly detection reduce the frequency of false positives compared to traditional methods?
Danielson: AI/ML techniques can be developed to detect common data quality issues from patient-attributable data from devices, common in Health IT, helping prevent inaccurate patient records. AI/ML techniques can also be developed to detect anomalies associated with the devices themselves, and with adequate training data, reduce false positives that would otherwise have been flagged for follow-up investigations, disruptive to normal clinical and security operations. AI/ML techniques can also be developed to begin to predict device failures, prior to them failing, and causing a resulting operational disruption.
In what ways can healthcare organizations balance the need for security with the need to maintain patient data privacy and accuracy?
Danielson: Healthcare organizations can balance the need for security, with the need to maintain data privacy and accuracy, by implementing a variety of security measures, including, but not limited to encryption of data in transit and at rest, firewalls to prevent unauthorized access to networks and data, spam filters to block malicious emails, web filters that can prevent staff from accessing malicious websites, antivirus solutions, role-based access control (RBAC), virtual private networks (VPNs), systematic security audits, system monitoring applications, multi-factor authentication, employee training, data classification, privileged account access management, and cloud security innovations.
How does the rise in healthcare data breaches underscore the need for more sophisticated security solutions and what role does AI play in enhancing the accuracy and consistency of patient data management?
Danielson: The rise in healthcare data breaches in an increasingly complex data environment is not surprising, as malicious actors are also leveraging emerging technologies to optimize their tactics. AI/ML can play a role in enhancing and automating security solutions to improve defenses
AI/ML can also play a role in enhancing the accuracy and consistency of patient-attributable data by employing an increasingly sophisticated set of data quality checks, as data is aggregated from a growing number of new sources
What are the key features of AI and ML algorithms that make them superior to traditional anomaly detection methods?
Danielson: Compared to traditional methods, machine learning solutions for anomaly detection enhance operational efficiency/performance as they process new data, show a lower rate of false positives with appropriate training data, and better deal with new types of anomalies, identifying outliers requiring new insights and interventions.
About Sarah Danielson
Sarah Danielson, MS, MBA, CPDHTS, CDH-L, FACHDM is the Sr Director of Strategy & Advisory, Health Solutions Group at Trace3. Sarah Danielson has served the healthcare sector on technology initiatives for over 20 years. She is passionate about advancing technology that improves security, resiliency, quality, value, patient safety, experience, and outcomes in the healthcare ecosystem.