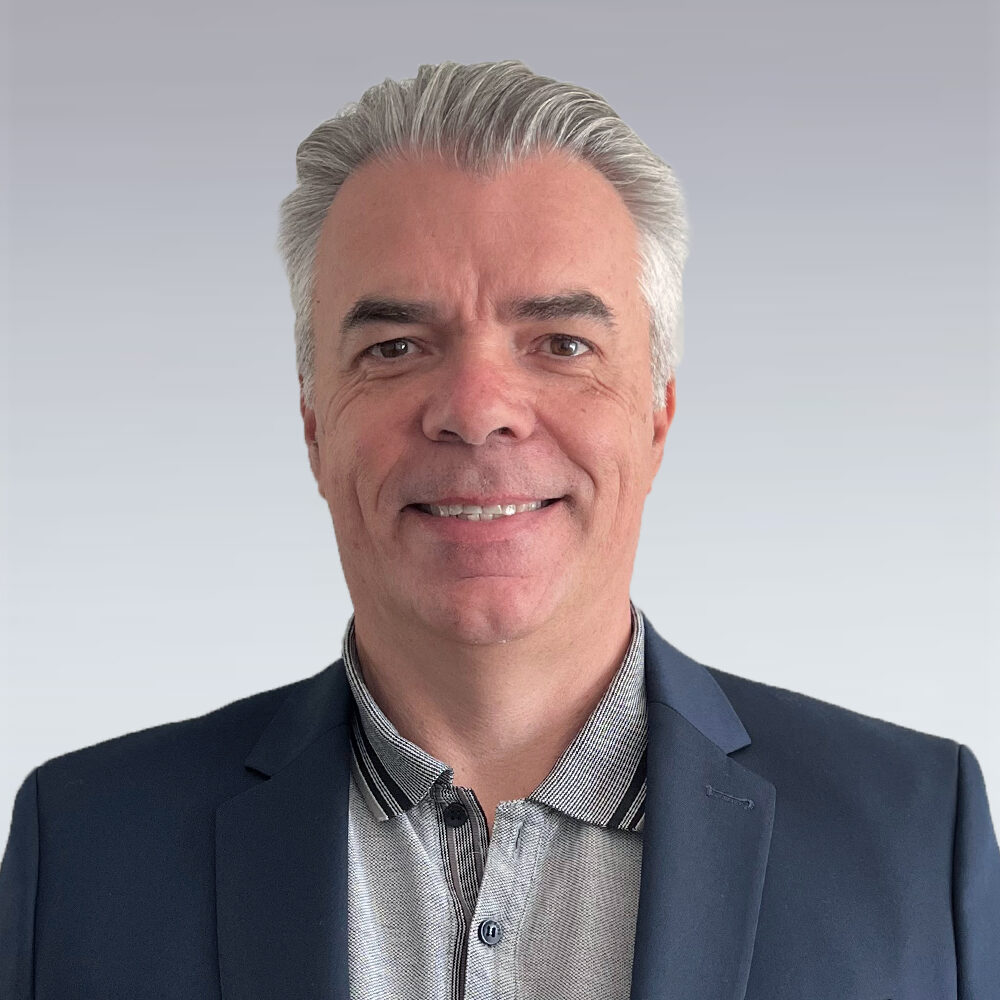
Artificial intelligence (AI) can be a sensitive subject for some healthcare professionals, and rightfully so. It was not too long ago that IBM’s Watson supercomputer won on “Jeopardy!” The hype and hubris that followed led to one of the great all-time examples of technology that some might say over-promoted and under-delivered. However, in the years that followed, AI, such as machine learning (ML), has managed to integrate into all facets of healthcare research and analysis. Some of the resulting breakthroughs are beginning to transform the way new therapies are developed and diseases are treated.
That phenomenon is having a huge impact in the field of neurology. Due to the unique nature of the field, and the largely unstructured manner in which patient data and disease progression are coded and tracked by clinicians, AI has become vital to unlocking insights that were never before possible.
Cracking the Code on Multiple Sclerosis
Looking at multiple sclerosis (MS) as an example, the three-character ICD-10-CM code, G35, is the only industry standard identifier universally used by clinicians to identify the condition. Unlike more structured datasets, such as those that are commonly found in ophthalmology, where—for example—a single condition like diabetic retinopathy will have 360 different ICD-10-CM codes tracking all manner of disease severity and progression, the neurology dataset is incredibly subjective. It does not take into account the myriad of symptoms, details about disease severity or progression or subtypes, or the unique patient journeys that real-world patients experience. Instead, the detailed experiences of patients with MS are largely captured in unstructured clinical notes that are keyed into the free text notes section of electronic health records (EHRs).
Utilizing standard coding, like the ICD-10 system, and even alternative clinical terminologies such as SNOMED-CT, to identify the existence of a condition, is an important datapoint. It defines the “what” of a disease. But for many complex conditions, such as MS, understanding the next level of detail helps to contextualize the “why” around a disease. Unlocking the “why” behind the diagnosis or treatment are areas where advanced AI techniques can provide a huge spectrum of valuable information around the disease manifestation and progression.
Until very recently, attempts to extract these types of meaningful insights from unstructured datasets were only achievable through labor-intensive, manual searches in which teams of analysts with enough clinical expertise to understand and interpret clinical notes—often nurse practitioners or doctors in training—would pore over several entries searching for clues. The model was not particularly efficient and not at all scalable. Worse, the data would become stale the minute the manual extraction was completed.
A Data-Driven Approach to Clinical Development
AI-powered large language models have helped to address these issues. With ML algorithms, it is possible to train models to continually track and analyze EHR data for hundreds of thousands of patient encounters to extract key information from clinical notes. Perhaps, more importantly, by carefully curating that data to flag keywords and patterns of language consistent with certain clinical cues, it has become possible to develop a new data-driven taxonomy for understanding neurological disease progression, treatment and outcomes.
Today, clinical development teams at the world’s leading life sciences companies are using these algorithms to instantly scour thousands of de-identified patient records to categorize the primary MS disease subtypes—relapsing-remitting MS (RRMS), secondary progressive MS (SPMS) and primary progressive MS (PPMS)—along with two common precursors to MS, clinically isolated syndrome (CIS) and radiologically isolated syndrome (RIS), by parsing data that was previously buried in EHR clinical notes. The process works by codifying language commonly used to describe these various disease progression milestones, such as “patient experiencing relapse” or “patient started using walker,” with real-world patient experiences to create a standard set of benchmarks.
By tracking these variables over time, and combining them with treatment information and patient encounter data, researchers are developing a clear, data-driven picture of disease progression and patient experience that inform a more targeted approach to treatment, clinical development and health economics and outcomes research (HEOR) strategies.
Believe the Hype
This approach is currently being deployed in all areas of neurology. With Parkinson’s disease, for example, researchers are now able to efficiently extract the cardinal signs of disease progression from hundreds of thousands of individual clinical notes. For Alzheimer’s disease, researchers are able to identify key variables from neurological tests and cognitive function exams that may hold the clues to future treatments.
Admittedly, these real-world use cases for AI in healthcare are not quite as simple as the bold pronouncements we heard a few years ago that AI would cure cancer. However, over time, the technology has made it possible to unlock new insights and dramatically speed the clinical research process, driving faster drug development and better therapies.
About Lawrence Whittle
Lawrence Whittle is president of Verana Health. Lawrence is responsible for driving market positioning and customer success. His remit spans a multitude of stakeholders, leading the healthcare clinician experience, medical society engagement, and life sciences collaborations, as well as the marketing and quantitative sciences functions to elevate awareness and value across the care continuum.
Lawrence has 25+ years experience as a senior executive within technologies companies spanning different industries, including Data Analytics and Life Sciences. His deep expertise in commercialization has covered elevating company positioning, driving sustainable customer value through deep in-market partnerships, and nurturing strong collaborations across all key functions of the business. His experience in early- and mid-stage companies has resulted in two IPOs and two M&A transactions.