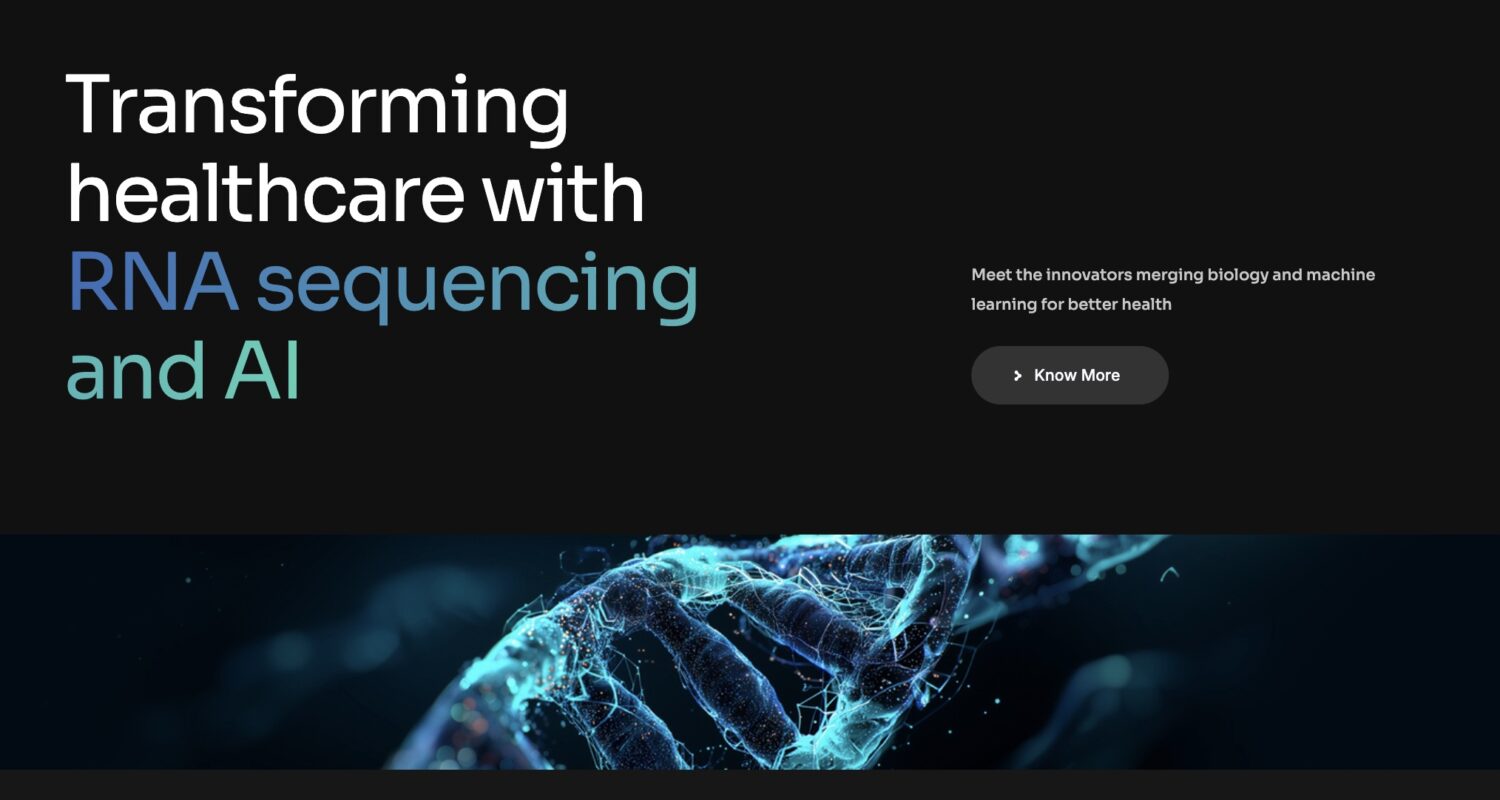
What You Should Know:
– Biostate AI, an innovator at the intersection of artificial intelligence and RNA sequencing raises $12M in Series A funding spearheaded by Accel, with participation from Gaingels, Mana Ventures, InfoEdge Ventures, and existing investors Matter Venture Partners, Vision Plus Capital, and Catapult Ventures. This brings the company’s total funding to over $20M.
– The newly acquired funds will be pivotal in advancing Biostate AI’s mission to unlock affordable and integrated precision medicine, beginning with the widespread accessibility of RNA sequencing (RNAseq) services for US-based molecular research.
– The company aims to develop clinically relevant predictive models, laying the groundwork for truly personalized therapeutics.
Unlocking the Transcriptome: A New Frontier in Precision Medicine
Founded by former professors and repeat entrepreneurs David Zhang (CEO) and Ashwin Gopinath (CTO), Biostate AI operates on the principle that the entire RNA transcriptome – the full range of RNA transcripts in a cell – is an underutilized real-time biomarker for human health. Until now, the comprehensive and simultaneous analysis of all these transcripts has been hampered by significant cost and analytical barriers. Biostate AI aims to eliminate these bottlenecks, envisioning a “one-stop shop” for precision medicine by making RNAseq significantly cheaper and more effective.
Overcoming Traditional RNAseq Limitations with AI and Innovation
Conventional RNA sequencing faces several key challenges that Biostate AI is engineered to solve:
- High Cost: It’s expensive, limiting the scale of research for many labs, especially as research budgets tighten. Biostate has developed patented biomolecular technologies (BIRT and PERD) that reduce the cost of turning tissue samples into RNAseq data by nearly an order of magnitude, effective on both fresh and decades-old tissues. This allows researchers to process 2-3 times more samples within existing budgets.
- Data Aggregation Issues: Combining datasets from various research sites often introduces “batch effects” – noise that can obscure subtle clinical signals. Biostate’s lower internal costs facilitate the collection of millions of consented, de-identified RNAseq profiles globally, creating a massive dataset to train sophisticated generative AI models.
- Lack of Standardization & Vendor Siloing: Inconsistent methodologies across studies make data comparison difficult, and reliance on multiple specialized vendors leads to communication breakdowns and slower workflows. Biostate’s unified workflow standardizes experiments, enabling its AI to consistently learn the “grammar of biology” without confounding batch effects. This also allows for the extraction of meaningful signals from smaller, clinically labeled cohorts to fine-tune models.
Towards General-Purpose AI for Understanding and Curing Disease
While Large Language Models learn from text, Biostate’s AI models identify gene expression signatures correlated with specific disease states and treatment responses. This enables the detection of subtle molecular changes that may precede clinical symptoms by weeks, months, or even years, facilitating earlier intervention.
“Rather than solve the diagnostics and therapeutics as separate, siloed problems for each disease, we believe that the modern and future AI can be general purpose to understand and help cure every disease,” said David Zhang, co-founder and CEO of Biostate AI, and former Associate Professor of Bioengineering at Rice University. “Every diagnostic I’ve built was about moving the answer closer to the patient. Biostate takes the biggest leap yet by making the whole transcriptome affordable.”
Early Traction and Future Expansion
The AI developed from this wealth of RNAseq data is intended to better inform clinicians of optimal treatment decisions. Biostate has already achieved internal proof-of-concept success in predicting disease recurrence in human leukemia patients and plans to expand collaborations with clinical partners in oncology, autoimmune disease, and cardiovascular disease.
Since commercializing its offering just two quarters ago, Biostate has processed RNAseq for over 10,000 samples from more than 150 collaborators and customers at leading institutions, including pilot projects for leukemia with Cornell and multiple sclerosis with the Accelerated Cure Project. The startup has also secured agreements to process several hundred thousand unlabeled samples annually, rapidly accelerating its dataset growth and AI development capabilities.