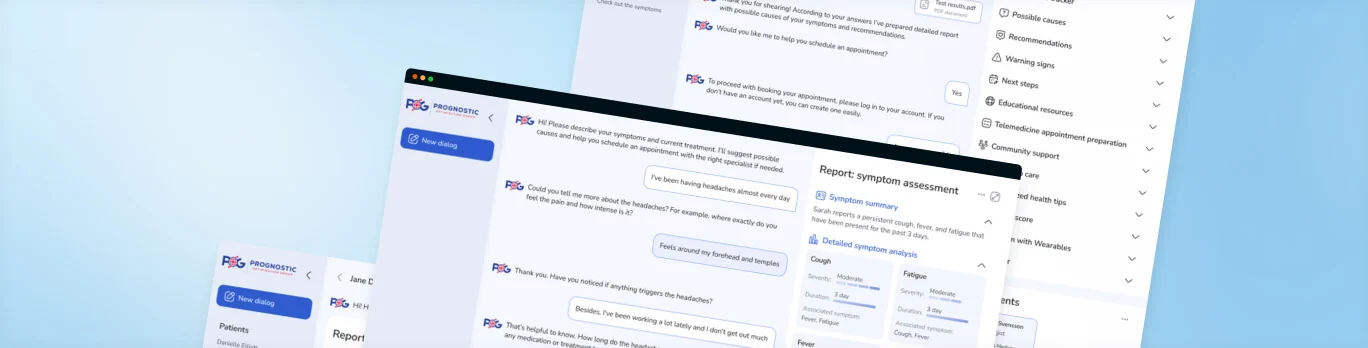
The American Cancer Society predicted 2 million new cancer cases in the US alone in 2024, which amounts to roughly 5,480 diagnoses every day. What makes matters worse is that the pandemic delayed care for more than 75% of cancer patients. Unsurprisingly, healthcare providers are turning to new technologies like AI in search of solutions. To gauge the magnitude of this trend, consider this: as of August 7, 2024, 950 AI/ML-enabled medical devices have received clearance from the FDA, with 76% of these designed for radiology. I also have observed a growing demand for AI in cancer diagnostics and treatment among healthtech startups, R&D companies, and laboratories.
I’d like to zoom in on three practical applications AI in oncology: medical chatbots for preliminary symptom assessment, AI-driven cancer imaging, and personalized treatment planning. I will share examples of tools, development, and ML model training recommendations. I will also examine where AI can realistically add value and where further advancements are needed.
Spotting Early Signs: Medical AI Chatbots for Preliminary Cancer Diagnosis
The burning question for companies considering this direction is: Can a general-purpose bot really diagnose cancer? The short answer is no—or, at least, not yet.
There was a study where ChatGPT-3.5, a general-purpose large language model (LLM), was asked about different cancer types and their treatment. The results showed that LLMs can sometimes mix correct and incorrect answers. Imagine if we tried to apply the model to actually diagnose cancer. At the same time, tools combining multiple models that have been trained on specific health datasets are entering the market. Examples include Microsoft’s Azure Health Bot and IBM’s watsonx Assistant. The next-generation models behind these products integrate knowledge from credible sources of medical information such as the US National Library of Medicine, CDC, and FDA. They might be promising for triage, although they still lack the nuance to factor in a patient’s complete medical history and lifestyle—elements that a human clinician would naturally consider. Therefore, a doctor’s examination remains a must.
Considering this, it is safe to say that, for now, chatbots cannot provide medical advice regarding such complex conditions as cancer. A more realistic use case is collecting symptoms and connecting users with healthcare providers based on the potential risks without recommending any treatment or medications.
The next logical question is: Should health-tech startups rely on these ready-made platforms or develop proprietary algorithms? Creating a bot tailored specifically to diagnosing cancer doesn’t seem feasible, and considering the scope of diseases and symptoms a general-purpose bot would need to cover, building and training a custom model would take years. So, I recommend sticking to platforms leveraging pre-trained models. They allow for faster deployment while aligning with health data protection standards and regulations. For instance, for one of our clients, our team created a fully HIPAA-compliant AI chatbot PoC using Azure Health Bot. Based on the reported symptoms’ assessment, it recommends self-monitoring, seeking emergency care, or scheduling a doctor’s appointment. If the last option is chosen, the bot suggests a list of available providers from integrated telehealth platforms such as Teladoc Health, Amwell, Doctor on Demand, MDLive, or Doxy.me.
A big challenge for medical chatbots is accurately interpreting human input, especially when it comes to subjective things such as symptom perception. It’s best to offer pre-built answer options like scales with examples of symptom intensity to prevent misinterpretation. The context of the reported symptoms can also play a key role in their analysis. Follow-up questions can give AI a clearer picture of the patient’s state. For example, if a patient reports fatigue, the bot can ask if it has started recently or been lasting and if it has been affecting them during a particular part of the day or throughout the whole day. Such questions help increase the accuracy of preliminary symptom assessment.
From Pixels to Progress: The Impact of AI in Cancer Imaging
AI-supported image analysis tools assist in analyzing X-ray images, mammograms, sonograms, MRI scans, and tissue slides. 723 radiological AI-powered tools have been FDA-cleared to this day, and the number keeps growing by 100 every year! Trained on numerous images, AI can help identify the subtlest details the human eye might miss.
In a recent project, our team built a desktop app for an ML algorithm that aids researchers and diagnosticians in interpreting test results for early lung cancer detection. Developed by bioAffinity Technologies, it is used in a non-invasive diagnostic lung cancer test CyPath® Lung. Connected to a flow cytometer, the algorithm analyzes cellular events within the patient’s sputum sample. bioAffinity’s proprietary solution showed 92% sensitivity and 87% specificity in identifying lung cancer in high-risk patients with small pulmonary nodules less than 20 millimeters.
Developing a custom ML model for cancer imaging solutions is a more realistic option today, as it’s difficult to find a pre-trained model that would fit a specific use case. Two factors impact the success of the model training. The first one is the quality of the training dataset, which depends on how large the dataset is and whether it includes data from patients of different age groups, genders, and ethnic backgrounds. Hospitals building medical image analysis software for internal use can opt for their own patient data. Of course, it must be anonymized before feeding it to the model to avoid any PHI breach risks. Public repositories, such as The Cancer Imaging Archive (TCIA), are also valid data sources. If the data is still insufficient, some simple data augmentation techniques can be used. For instance, rotating, cropping, and adjusting contrast can help artificially expand the dataset and imitate variations in real medical images.
The second factor is the accuracy and completeness of image annotations. Those are essential because they teach the model what to look for in each image. As my colleagues iom data science say: “If you teach the model that a car is a horse, it will inevitably mark every car as a horse”. That’s why you need to involve medical professionals to label the training images as precisely as possible. With oncologists’ guidance, data scientists use annotation tools to carefully outline a tumor’s boundaries and identify its unique shape, texture, and other defining characteristics. Detailed annotations help the model learn to distinguish between normal and abnormal tissue more accurately.
A New Era in Cancer Treatment Planning
Although most of the AI tools created for treatment planning are still R&D initiatives, they may considerably impact cancer care in the future. One project in this area I’ve been interested in is Personalized single-cell Expression-based Planning for Treatments in Oncology, or PERCEPTION.
Using PERCEPTION, scientists get detailed information from individual cells to study how tumors are structured, how they change over time, and how they might become resistant to treatments. Potentially, this tool can help clinicians timely adapt to the evolution of cancer cells and even modify treatment strategies.
Recently, three independent clinical trials have been published where the tool successfully predicted the response to monotherapy and combination treatment for myeloma, breast, and lung cancer. In each case, PERCEPTION accurately sorted patients into two groups: those who responded well to treatment and those who did not. In lung cancer cases, it even tracked how some patients formed resistance to the drugs as their disease progressed.
The creators say that PERCEPTION is not ready for implementation in hospitals yet. Indeed, even 80–90% accuracy of ML models may be insufficient in healthcare. Even a tiny percentage of erroneous decisions can significantly harm patients. However, this is a promising direction in which AI can bloom as technology progresses.
Prospects of AI in Cancer Care
AI has come a long way, from automating paperwork to diagnosing cancer. Its growing role in cancer diagnostics sets the stage for a broader application across the oncology field. As both patients and clinicians are getting more comfortable with it, AI is moving towards conquering other areas of cancer care, such as precision medicine and treatment personalization.
About Gala Batsishcha
Gala Batsishcha is a healthcare IT consultant at ScienceSoft. She helps startups launch innovative healthtech products, navigating compliance requirements and promoting accessibility. She also works with healthcare organizations to ensure a personalized approach to patient care with a focus on security. Gala believes in the power of learning and is always eager to share her knowledge and experience.