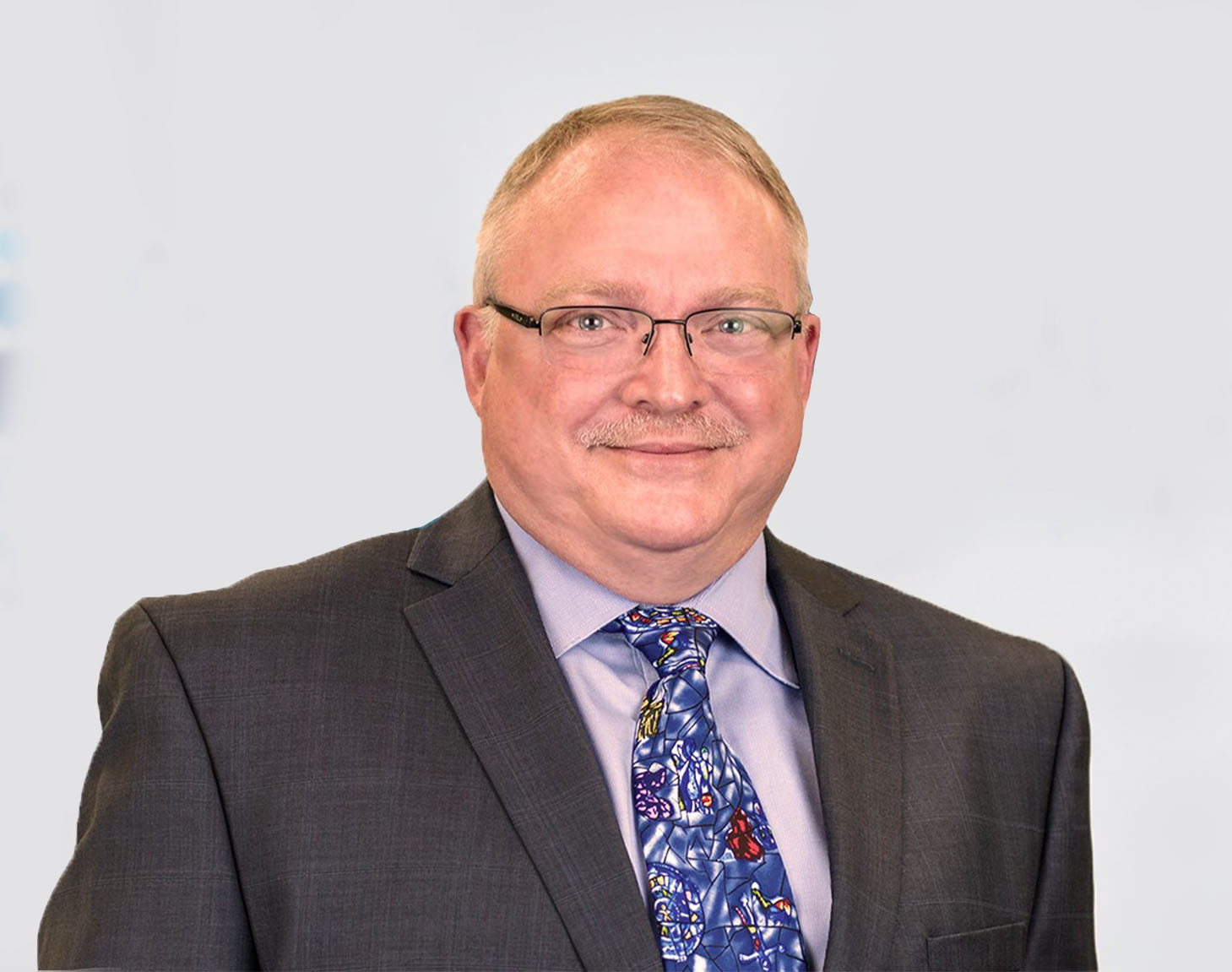
Healthcare is on the cusp of a transformative era, with artificial intelligence (AI) and large language models (LLMs) demonstrating remarkable potential in streamlining clinical documentation, quality measurement, and medical coding. As these technologies mature, forward-thinking healthcare provider organizations are exploring ways to leverage AI to improve data quality, reduce clinician burden, and ultimately enhance patient care.
AI’s Role in Clinical Documentation and Coding
AI, through natural language processing (NLP) and large language models (LLMs), has the potential to revolutionize clinical documentation and coding by accurately extracting clinical concepts from patient encounter notes and conversations. By training on vast medical texts, AI models can identify relevant codes and quality measures, streamlining documentation, billing, and compliance processes. This technological advancement not only boosts efficiency but also enhances the accuracy of medical records and billing, leading to improved healthcare management and patient care outcomes.
AI can also suggest investigating relevant conditions based on patient symptoms, such as associating “shortness of breath at night” with sleep apnea, heart failure, or COPD for hierarchical condition category (HCC) risk adjustment. This capability to link free text with standardized diagnostic codes and terminology supports clinicians in comprehensive care documentation. The next step is to develop tools that allow clinicians to diagnostically filter codes and terminologies, identifying pertinent information for any patient condition or diagnosis with a simple click or voice command. In a recent study at the Mount Sinai Icahn School of Medicine, researchers found that LLMS are not suited for accurate medical coding. The authors said that “While GPT-4 performed the best in the study with the highest exact match rates for ICD-9-CM at 45.9%, ICD-10-CM at 33.9% and CPT codes at 49.8%, ‘unacceptably large’ errors remained.”
The HTI-1 Final Rule: A Step Towards Transparency
The Office of the National Coordinator for Health IT (ONC) has taken a significant step towards algorithmic transparency with the recently released Health Data, Technology, and Interoperability (HTI-1) Final Rule. This groundbreaking regulation establishes transparency requirements for AI and predictive algorithms used in certified health IT, ensuring that clinical users can access crucial information about the algorithms supporting their decision-making processes.
The HTI-1 Final Rule introduces groundbreaking transparency requirements for AI and predictive algorithms in certified health IT, impacting a sizable portion of the healthcare industry. EHR/EMR vendors must provide clinical users with consistent, baseline information about the algorithms used in decision-making, enabling users to assess algorithms for fairness, appropriateness, validity, effectiveness, and safety (FAVES).
To comply with these requirements, vendors must demonstrate the algorithms’ fairness and lack of bias, clearly communicate intended use cases and limitations, document data sources, models, and performance metrics used in algorithm development and validation, provide evidence of real-world effectiveness in improving patient outcomes and clinical decision-making, and implement safety monitoring and reporting systems with guidance on appropriate use and interpretation of algorithm outputs.
Meeting these requirements will require substantial investments from vendors in documenting, testing, and validating algorithms, creating user-friendly interfaces and educational materials, and establishing ongoing processes for monitoring real-world performance, addressing issues, and updating user information.
Challenges in AI-Driven Clinical Decision-Making
While AI and LLMs show immense promise in extracting clinical concepts from patient narratives and suggesting relevant diagnostic codes, there are still significant challenges to overcome. One major limitation is the lack of representative data that captures the unique needs and clinical presentations of minority groups, which can lead to biased algorithmic outputs. Additionally, the complexity of medical language and the fragmented nature of patient records pose barriers to the seamless integration of AI in clinical decision-making.
To realize the full potential of AI in healthcare, it is essential to prioritize data quality and develop robust explanatory frameworks for AI-driven decision support. Healthcare provider organizations must invest in collecting representative data, validating AI suggestions, and integrating these tools into existing clinical workflows in a user-friendly manner. By combining AI text analytics with expert systems and clinical validation, advanced clinical intelligence solutions can help reduce documentation burdens for clinicians while optimizing the capture of quality measures and risk adjustment factors.
Collaboration is the Key to Success
As the industry navigates this new landscape,the collaboration between healthcare providers, AI developers, and policymakers will be crucial. By working together to establish best practices, address potential challenges, and ensure algorithmic transparency, the healthcare community can harness the power of AI to improve data quality, reduce clinician burden, and deliver better patient care.
The path forward requires ongoing advances in data collection, algorithm development, and human-AI partnerships. By deploying AI responsibly and equitably, healthcare organizations can unlock the transformative potential of clinical intelligence, paving the way for a future where data-driven insights and streamlined processes empower clinicians to focus on what matters most – providing the best possible care for their patients.
About Jay Anders, MD
As Chief Medical Officer of Medicomp Systems, Dr. Anders supports product development, serving as a representative and voice for the physician and healthcare community. He is a fervent advocate for finding ways to make technology an enabler for clinicians––rather than a hindrance. He is also the host of the award-winning HealthcareNOW Radio podcast, “Tell Me Where IT Hurts,” which explores the topics of physician burnout, EHR clinical usability, healthcare data interoperability, and the evolving role of technology in healthcare with a variety of industry experts and pundits.