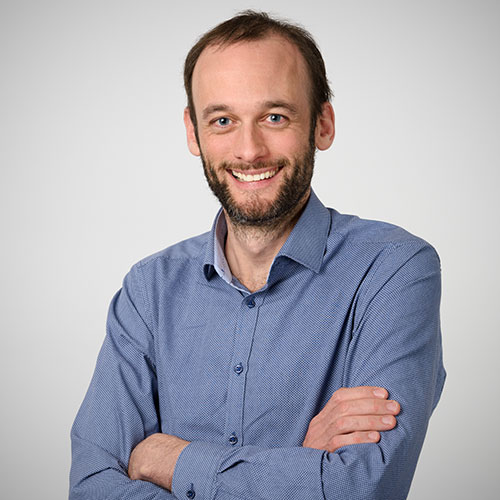
Forecasting the influence that artificial intelligence (AI)/machine learning technologies will have on the future of healthcare has created a cottage industry in the hype. From the overzealous aspirations of IBM’s Watson Health initiative to the inclusion of AI on Gartner’s 2021 Hype Cycle at the “Peak of Inflated Expectations,” so much noise has been made around AI/machine learning in healthcare that it can be difficult to appreciate the current impact of these technologies on drug discovery, clinical research, and other aspects of pharmaceutical R&D.
AI is a broad umbrella term simply meaning machines, particularly computers, that perform tasks typically conducted by humans, such as autonomously driving a car, scanning through reams of contracts and legal documents, translating speech to text or identifying faces in a crowd. The branch of AI used most commonly today in drug discovery and development is called machine learning. Machine learning systems are trained to comb through large amounts of existing data to detect patterns, predict outcomes, and make decisions with minimal human intervention.
The challenge for those working in and watching this fast-moving space is separating the breakthroughs that have real power to transform the industry from the hype that has the power to destroy it. While hype is a natural part of the evolution of any new technology, in healthcare it has created an unfortunate scenario in which many of the stakeholders who stand to benefit most from innovation have become the most skeptical of its potential.
Examples of this problem occur when exaggerated, too-good-to-be-true headlines, like “First Wholly AI-Developed Drug Enters Phase 1 Trials,” imply that robots have taken the helm and are creating cures using truly generative AI. In fact, what’s really happening is that machine learning is helping to drive incremental advances around known chemical structures. But, when the headlines eventually fall short of the sci-fi fantasies created in the press, the entire space loses credibility. Ultimately, this could undermine existing projects and slow future momentum by cooling investor sentiment and discouraging new innovation.
At this crucial moment in the evolution of drug discovery, it’s important to recognize and communicate that while machine learning is impacting key aspects of R&D, the technology is not performing the work independently.
The AI/machine learning technologies currently having the most significant impact on R&D are not autonomous compound creation machines. They are workhorses that are helping human researchers dig deeper, identify new disease-relevant proteins faster, and unlock details of molecular structures that would have been impossible to see even five years ago. For example, at HotSpot Therapeutics, we are using these technologies to home in on “natural hotspots,” which are the previously unreachable pockets on proteins that act as endogenous on/off switches.
Addressing the Limitations
Based on our work in this space, we’ve had a front-row seat to not only the strengths of AI and machine learning but also their limitations. Chief among these are incomplete data and lack of complexity in the data sets used to train the models. Machine learning is only as good as the data on which it relies. Rough estimates indicate that 1060 drug-like molecules are theoretically possible, which is comparable to estimates of the number of atoms in the universe. Therefore, it’s impossible to capture the vastness of chemical space solely with machine learning when less than 200 million drug-like molecules have been synthesized and characterized by humans. The goal in drug discovery is to extrapolate away from the molecules already synthesized, but machine learning models can, by definition, only be effectively used to predict molecules highly similar to those molecules used to train the model. The reliability of the predictions quickly breaks down as the molecules become dissimilar.
Improving reliability can be achieved in a number of ways. For example, in our work identifying and drugging “natural hotspots,” the privileged pockets on proteins that act as endogenous on/off switches, we are able to use data mining tools and machine learning algorithms to better understand the 3D footprints of cells. This approach allows us to spot similarities and patterns of behavior to isolate specific biological and chemical “zip codes” where we can focus our research, rather than trying to analyze each molecule individually. It’s an amazing leap forward for efficiency, but hardly the robotic magic depicted by popular culture. The computer must be paired with humans that have a deep understanding of the problem at hand and a true appreciation of the power and limitations of machine learning.
Demystifying the Hype
Until now, much of the discourse around AI in healthcare has ignored those decidedly practical functions in favor of sensationalized depictions of cool new AI-based tech. Ironically, that hype has done more harm than good by questioning the credibility of the technology and limiting the full potential of AI-driven advances.
It is our job as scientists to be precise and honest in describing what AI and machine learning entail, where the biggest impacts can be made, which additional technologies are required to enable their functioning, and where they simply aren’t the right tools for the problem. In short, we need to bring a level of intellectual honesty to machine learning (and really all technology) before we can move past the hype and embrace the hope.
About Jonathan Montagu
Jonathan Montagu is President and Chief Executive Officer at HotSpot Therapeutics, a biotechnology company pioneering the discovery and development of small molecule allosteric therapies for the treatment of cancer and autoimmune diseases.