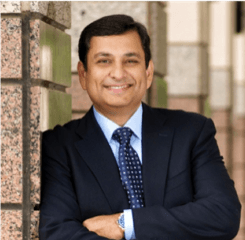
While the public perceives artificial intelligence as futuristic, AI is already intertwined with healthcare today. As a PwC report observed, AI is transforming numerous aspects of healthcare, from medical training and research to wellness and treatment. But while AI is already supercharging our capabilities (it’s 30 times faster and 99 percent accurate when reviewing mammograms, reducing the need for unnecessary biopsies, for example), AI is also supercharging the disparities that are baked into our healthcare system.
The issue, however, isn’t a question of technology, it’s a matter of transparency and trust. Trust is the foundation of digital systems. Without trust, AI cannot deliver on its potential value. To trust an AI system, we must have confidence in its decisions. Reliability, fairness, interpretability, robustness, and safety will need to be the underpinnings of Health AI.
One area that provides a useful case study for the importance of trusted AI systems is patient intake. Hospitals nationwide have already deployed AI to triage patients in order to make better use of medical resources and ensure appropriate care is delivered in a timely manner. But the inputs AI requires doesn’t come from a vacuum, and in some cases, our existing biases are integrated into the AI’s decisioning.
Consider a recent report in The Wall Street Journal about a hospital intake algorithm that exhibited racial bias. The algorithm gave healthier white patients the same ranking as black patients who had much worse chronic illnesses, as well as poorer laboratory results and vital signs. As one of the researchers who discovered the problem explained, “What the algorithm is doing is letting healthier white patients cut in line ahead of sicker black patients.”
How could this happen? Turns out, the algorithm used cost to rank patients for intake. Because spending for black patients was less than for white patients with similar medical conditions, the AI inadvertently gave preference to white patients over black patients. Put it another way, the AI exacerbated racial disparities that are already present in our healthcare system.
But bad outcomes aren’t inevitable, even though the AI’s textbook is an imperfect world. As another researcher who helped discover the intake problem pointed out, an alternative algorithm could actually decrease racial disparities. In fact, those researchers created an alternate algorithm that increased the percentage of those identified for extra help who were black to about 47 percent, up from 18 percent. Nevertheless, given the enormous power of AI, it’s important to ask how we can build better systems from the start?
First, we must understand that AI isn’t like other tools we’ve developed throughout human history. The technology is a thinking partner, one we need to understand, and ultimately trust. That process isn’t automatic, nor is it inherently transparent. Understanding and trusting an AI is akin to understanding and trusting complex human institutions. As with those institutions, we can build-in principles that ensure trust. A trusted AI must adhere to five principles:
1. Data rights: Do you have the rights to the data and is the data reliable?
2. Explainability: Is your AI transparent?
3. Fairness: Is your AI unbiased and fair?
4. Robustness: Is your AI robust and secure?
5. Compliance: Is your AI appropriately governed?
These five principles underscore the need for a more human-centric approach to integrating AI with healthcare. In laymen’s terms, by building healthcare AIs with these five principles in mind, doctors and patients have the ability to “look under the hood.”
While we’d never call racial preferences fair, we might not intuitively see that considering a factor as benign as the cost would lead to an unfair outcome. What that means is that fairness can’t live within a silo if you want to build a trusted AI. Instead, each principle works in conjunction with the other.
So, for example, fairness can only be properly understood if we first meet the threshold question of data access and then address explainability so that all stakeholders can comprehend the AI’s decisioning. Robustness and compliance must also come into play so that stakeholders can trust that the process hasn’t been tampered with and that there is a mechanism for human review so that we don’t unwittingly cede control of our lives to machines we don’t fully understand.
In the case of the problematic intake AI, doctors didn’t question the AI’s findings, although to be fair, those doctors may not have been given the tools to do so. Still, it’s an important lesson: AI must serve humanity, not the other way around. When doctors are empowered to challenge AI, they actually maximize their benefits.
Which is to say, the doctor still knows best because it takes humans to advocate for human-centric AI systems. So, as we deploy AIs throughout healthcare, we must educate doctors and patients about how they work, in order to avoid undesirable outcomes and build the trust needed to reap the long-term benefits of this new technology.