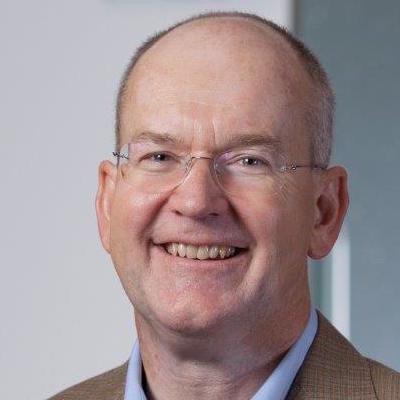
Payer-provider relations are undergoing a major shift. The classic adversarial battles in the fee-for-service world are gradually giving way to capitated plans with more win-win incentives. The reality is that providers and payers famously have to live in the “two canoes” world, with one foot in volume-based revenue and the other foot in value-based revenue.
As one can see, there is extraordinary information and calculation needed to optimize revenues on both sides. The good news is AI thrives in complex information landscapes where the number of variables to be considered is too large for a classic statistical model.
Top areas for payers and providers to leverage AI
Increasingly, the most important payment regimen to consider is Medicare Advantage (MA). MA is capitated and many of the lessons of payer-provider interactions from MA, will also likely extend into managed Medicaid. AI can support each phase of payment, including initial contracting, network design, preventative services for patients covered by the plan, actual care covered by the plan, and prior authorization.
Choosing patients based on calculations optimizing CMS’s Hierarchical Condition Category classification scheme may also be impacted by AI models. Today, MA plans are doing selective contracting and selective broker incentives based on estimates of expected actuarial risk. It is not known whether their prediction models contain AI, but it would be hard to imagine that plans wouldn’t consider an AI algorithm in targeting patient populations to serve.
Selecting providers in the network is usually a coarser activity, especially as the consolidation of delivery system providers has reduced the options for payer contracts. Based on that, AI could also play a role in the pricing of individual services in a network contract and the payment rate micro-incentives at the margin. Ultimately, pricing can be seen as a complex gradient fit computer science problem. Solving gradient fit on complex mathematical surfaces is the way many AIs work under the covers.
On the care side, one can look both at prevention and actual treatment. Prevention is very complex because there is little payment for early disease prevention, with almost all payments being for acute care. However, the plans that retain the same members for long periods of time are incentivized to provide prevention.
The specific preventative services that make economic sense in payer-provider contracts are evolving. The pressures for prevention are growing because circulating tumor cells and circulating free DNA to identify early cancers and modern plaque imaging predicting myocardial infarction and stroke up to a decade in advance will drive allocation of care. It is also likely that the “Make America Healthy Again” movement with its focus on prevention will change CMS payment priorities.
Meanwhile, most dollars are spent on acute care of chronic illnesses, where the opportunities for AI wins are arguably the greatest. This is because acute care patients generate large amounts of data across clinical notes, lab tests, and images — all of which can help predict the need for further care. There is even enough clinical data for large data models to move beyond today’s “Internet screen scraping” large language models (LLMs). At least one vendor is already building AI models using clinical data, as well as a large amount of claims data, to provide much higher accuracy in predicting outcomes and likely care compared to LLMs.
Actual diagnosis has always fascinated AI researchers. Direct diagnosis of general illness requires very high accuracy, and it’s not clear yet if any existing diagnostic AIs have that capability. AI is already embedded within EMRs to support services related to diagnosis, such as summarizing charts and assisted note-taking, and in the future might also drive chart reviews and alert functions.
The most contentious area of payer-provider relations, prior authorization, is another area where AI can be used. Payers can use AI to help determine which procedures and activities to enroll in prior authorization reviews, even before a request is made or based on provider performance or likelihood of approval. Then, once a provider has made a prior authorization request, payers can use generative AI to determine whether the specific service requested should be paid for.
Providers can also use AI to frame their responses to prior authorization queries. Any use of AI here would need to occur with the cooperation of the EHR vendors — since the workflows of ordering reviewable services occur in the EHR.
The Role of Data Interoperability in AI’s Emergence
One critical aspect of AI that has not been discussed as much is how to actually get the data to run the algorithms. This has two phases – the build phase and the run phase.
Federal policy, including the 21st Century Cures Act, has made application programming interfaces the law of the land. The Cures Act required EHRs to provide interfaces “without special effort,” or without proprietary disablement of data interoperability. CMS has a series of parallel data enablement rules, starting with a 2020 rule requiring CMS-regulated payers to provide APIs for patient apps and now with the 2024 CMS 0057 rule, requiring payers to provide similar APIs for providers and other payers.
The power of these APIs lies in their use of the FHIR (Fast Healthcare Interoperability Resources) API data transmission standard. This is the first data transmission format that allows for seamless modern computing at the heart of AI (the same APIs power every app on our smartphones). Prior efforts to access clinical data were complicated by proprietary APIs and the need to dig into bespoke databases and do field conversions. Now, database fields can be attacked with modern natural language processing techniques, but the information content of structured, codified data will still be higher than using unstructured data.
Another underappreciated part of AI in payer-provider interactions is how and where to store the underlying claims and clinical data. Today’s storage strategies are fundamentally inadequate to store and successfully employ AI on claims and clinical data. If you look at payers, their enterprise software record is typically a claims engine backed up with an enterprise data warehouse. For providers, their enterprise software record is the EMR. For larger providers that EMR may have backup with an OLAP database or an enterprise data warehouse. These data stores were historically designed for purely structured data accessible through SQL.
As pure relational database architectures make “big data” type of computing difficult, if not impossible, many “big data” enterprises are now moving to Lakehouse architectures. These combine data flexibility, massive horizontal scalability, and seamless computability in one single cloud environment. Increasingly, API-first business models for payers and providers will necessitate the move to such modern data architectures.
While payer-provider interactions have historically moved at the latency of the revenue cycle, which is on the order of a week or a month, modern interactions with patients and providers will need real-time or near-real-time APIs. This is the world that federal policymakers envision and what we expect and experience in every commercial interaction in our lives. Payer-provider interactions have escaped the requirement for immediacy thus far, but the winners in the future will have real-time performance as a center point of their strategy and part of almost every payer-provider interaction involving care. Real-time APIs are also central to harnessing AI in payer-provider interactions.
The AI and API Future Looks Bright
The payer-provider landscape is evolving as new policies and software opportunities continue to shape who the winners are. AI sitting on top of modern APIs and data platforms will certainly play an increasing and exciting part in improving healthcare in the United States.
About Donald Rucker, MD
Donald Rucker, MD is Chief Strategy Officer for 1upHealth and serves as an advisor to Genhealth.ai. Dr. Rucker was the National Coordinator of ONC.