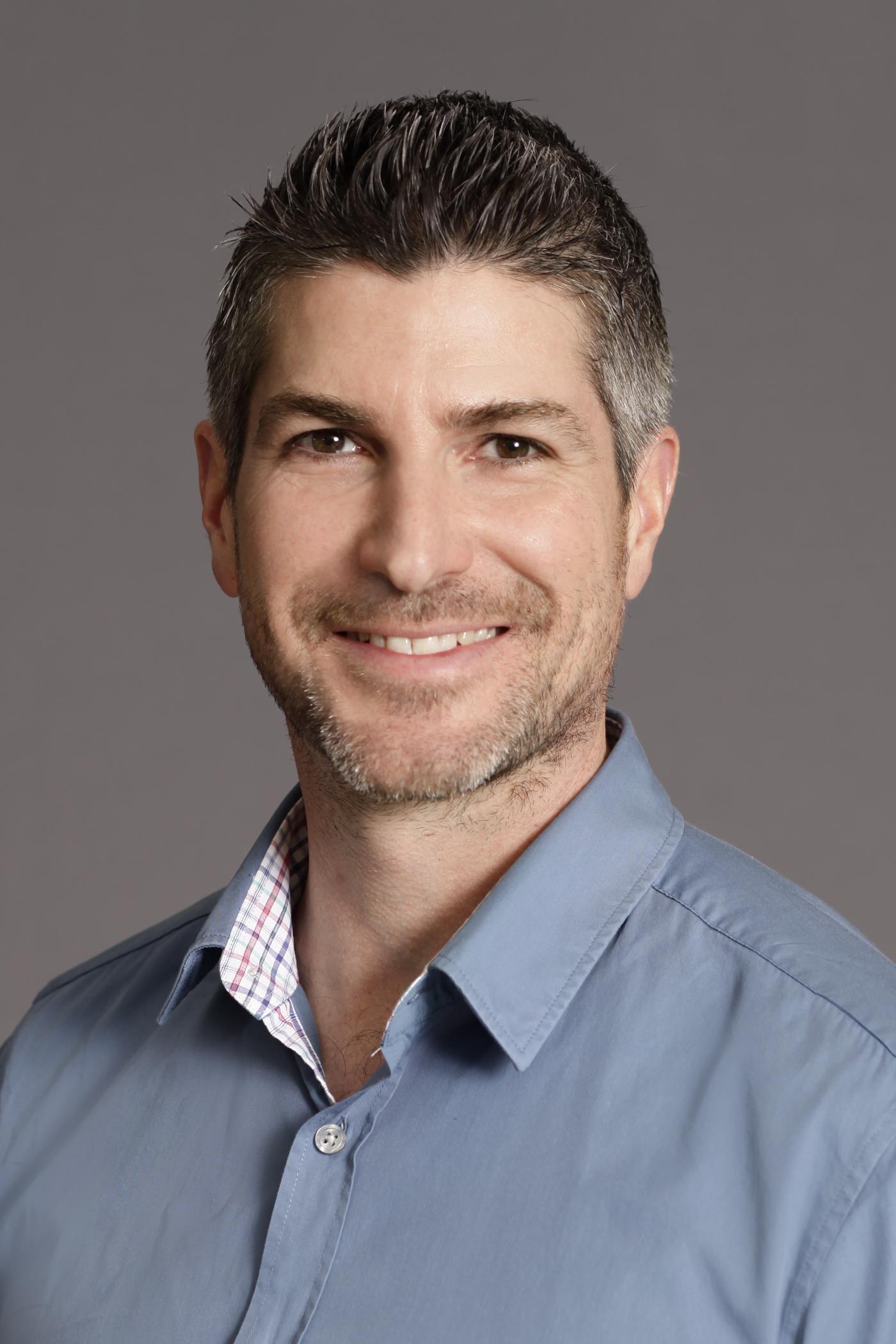
U.S. healthcare costs have skyrocketed over the last decade and show no sign of slowing down. We currently spend $3.5 trillion a year on healthcare—about $11,000 per person, more than twice the average per-person spending in other developed countries. And healthcare costs are expected to nearly double to $6 trillion by 2027, further adding to the financial burden on patients, plans, and providers.
Healthcare organizations are exploring a wide range of options to help contain costs while ensuring continued access to high-quality care. Value-based reimbursement models, among the most popular options, have been shown to lower costs by as much as 15.6% thanks to fewer emergency room visits and improved preventative care. However, high-risk patients still pose a major challenge.
Just 5% of patients account for nearly 50% of all healthcare costs. Early diagnosis and intervention are critical for improving the long-term health of high-risk individuals, as well as lowering their total cost of care. However, identifying preventive care opportunities for the right patients at the right time has traditionally been a challenge. Today, machine learning has emerged as a powerful technique to provide insights to clinicians and care managers that can help them spot health risks and intervene earlier.
The stakes are higher for high-risk patients
As providers feel the pressure to control care costs, high-risk patients pose a unique challenge. Comorbidities can complicate disease management, and many patients face preexisting conditions or a propensity to develop them. These individuals become frequent utilizers, develop complex medical conditions, and are sometimes noncompliant with treatment plans. They may also face social determinants of health (SDoH) issues as well, lacking the support or access they need to healthy lifestyle options that could help mitigate health issues.
As a result, high-risk patients require excess resources, complex monitoring, and additional treatment. They’re often not diagnosed early enough nor treated preventively, which means that once diagnosed, their conditions are harder and more expensive to manage. Consequently, the patients who need quality, affordable care the most often can’t afford it.
Machine learning can help solve the problem
These complex challenges call for innovative solutions. Machine learning models and predictive analytics can help providers and plans to identify high-risk patients before they develop costly care needs. By culling through individual and population data to identify risk factors, providers can work with patients to implement preventative care and early intervention strategies that can both contribute to better health and substantially reduce the cost of care. Here’s how it works:
– A data analysis platform ingests and analyzes structured and unstructured data from patient charts including clinical notes, diagnostic test results, patient health history, and more to get a clearer picture of each individual’s complete health profile.
– This health profile is run through machine learning models to identify risk factors for specific conditions or health complications. For example, an algorithm might look for a specific obesity indicator that correlates with the development of Type II diabetes, or patients with high blood pressure who may be at risk of a heart attack, stroke, or kidney disease based on clinical evidence.
– Based on this data, physicians and care managers can identify patients with the highest risk of developing a chronic condition and intervene early with the appropriate diagnostics, treatments, and support programs to prevent those issues from developing or progressing.
Because machine learning models can analyze large healthcare datasets at a speed, scale, and accuracy that no human could ever match, they can deliver these clinical insights quickly to inform real-time care decisions. For example, as data is entered into an EHR during a patient encounter, a physician may be prompted to screen for a likely health risk. Machine learning models can also be used to surface behavioral or social factors mentioned in encounter notes and sent to care managers to inform outreach and enrollments for SDoH programs.
These insights, available at scale via machine learning, can have a dramatic impact on plans and providers’ ability to deliver more effective, timely preventive care for high-risk patients. As a result, patient care costs will decrease and quality of life will improve.
Access to data is critical
In order to transform the way high-risk patients are diagnosed and treated using machine learning, health IT teams must have reliable access to patient data from multiple systems within their organization and elsewhere in the healthcare ecosystem. They must also have access to a longitudinal patient record that captures what’s happened to a patient’s health over time. Both types of data access require plans and providers to share more information with each other on a frequent basis.
Fortunately, big data platforms can be used to integrate data from disparate systems in a variety of formats. Pulling data from Electronic Medical Record Systems (EMRs) or using optical character recognition (OCR) to extract information from faxed or printed patient charts, teams can then deploy machine learning models to mine for high-risk patient indicators and extract usable new insights into patient and population health trends to improve care.
Given the difficulty of high-risk population management and the massive impact of high-risk spending, machine learning is the best solution to solving the problem of late patient intervention. By employing AI techniques, providers and plans can have an immediate, direct impact on bringing down the cost of care and help patients have an overall more effective, coordinated care experience.
About Mark Scott, Chief Marketing Officer at Apixio
Mark Scott has almost two decades of healthcare marketing and communications experience. He has overhauled and launched the global brands of two multibillion-dollar public companies: San Diego-based medical technology maker CareFusion and Massachusetts-based diagnostic device company Alere. While at Masimo, he launched the Patient Safety Movement which helped bring national attention to the issues of patient safety and garnered keynote speakers such as President Bill Clinton. Mark’s deep experience covers the complete marketing spectrum, including product marketing, launch activations, brand development, product positioning, and messaging, packaging and labeling, public relations, content marketing, website development, and crisis communications.