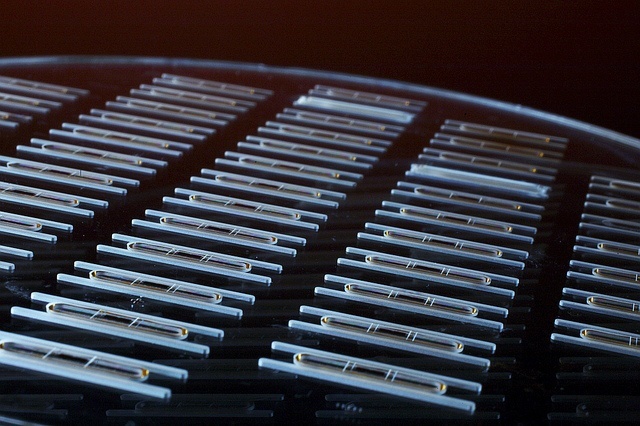
There’s a rising tide of healthcare data. It lifts many hopes for better healthcare, but also surfaces one troubling issue: reliability of data. Just how confident are you of the reliability of your data?
As a healthcare provider, you already know that data permeates your office workload. This impacts a critical feature of your operations: your workflow, a process you probably evolved over many years. Suddenly, you’re now doing “refreshes” to accommodate the new data volumes you’re seeing. “We’ve always done it this way” – that just does not cut it any longer.
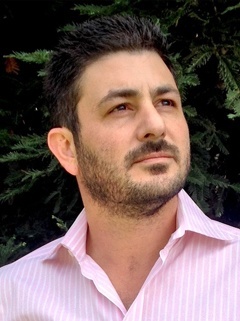
Time was when you had dictation, writing and paper records. You now have many data input options (EMRs, voice-enabled documentation and more).
So volume keeps growing, tools get more complex. Bigger yet are the issues around understanding your data, some not really obvious. For physicians, the EMR demands careful checks of patient records, new ways to capture care offered elsewhere, new diagnostic tools and ways for updating your patient’s condition — plus a bigger focus on “quality assurance.” Your “inputs” now need accuracy checks. It also means you’re the new data entry analyst on the block, and you’re burdened with an extra tall order for vigilance.
Now, how reliable is your data?
Example: At the point of care, as ICD codes get assigned to cases, there are some common errors, but their rates may top the 20% level (and higher still in some studies that have carefully assessed the data error issue). The inaccuracies may come from patient behavior, the record trail itself, the provider or physician. But errors do seep into the record: A physician uses a typical synonym to label ‘‘stroke’’: She can choose “cerebrovascular accident,” “cerebral occlusion,” “cerebral infarction” or “apoplexy.” Which is right?
Even if we correct for study differences among error-rate studies of clinical data, we know the error rates are unacceptable. The complexity of a case, provider inexperience, patients lacking skill in discussing symptoms, or the reluctance to listen to a patient’s view of her condition – all matter. It’s not uncommon to hear that even professional nurses may not be taken seriously as they describe their own condition to an emergency room provider.
An Experiment in Error Tolerance
If it seems like overkill to belabor the issues around data, picture this:
–Your child, driven from athletic field to an ER, being diagnosed for a traumatic hit to the head. Will all diagnoses work, and will you trust them?
–Your aging parent, living with multiple chronic conditions, uncertain about the new pains in her body. Where in her treatment steps will you overlook “understandable” error?
At moments of truth like these, we lose patience and tolerance for any (let alone “understandable”) errors. But medicine is still catching up with us. Clinical errors still can, and do create chains of miscues that prove fatal.
The quiet fact: while errors continue to crop up – at stubborn levels and rates — we know how to minimize them at the point of care. We know the “hacks” needed to produce much better data quality, and how to use those tools.
Nonetheless, data errors continue to get “spiraled” into the medical record and analytics trail. So when it’s time for analytics, there’s only so much that can be extracted as the “true record” of a patient meeting. By then, it’s too late. By that point, an inaccurate diagnosis and recording errors could well be compounded by the medications and treatment used.
Simple human error shadows many issues even with data missing from the picture. In the United States, remember, we still see more than 2,300 annual wrong-site, wrong-patient operations (about 46 per week). These may be “understandable”—but acceptable? Add data to this kind of picture, and it’s a volatile mix.
The healthcare system is beginning to tackle the healthcare data issue with some pace; however, the data remains fairly unreliable. We know U.S. medicine has many core issues, so while data quality gets mention, it does not attract follow-through. Healthcare, meanwhile, correctly still targets the triple aim (better care, better quality, lower cost). It responds to practical concerns: Expensive drugs (Sovaldi) or new policies (ONC on interoperability). But data quality issues live on and may well escalate.
For Interoperability to Work, Fix the Data Issues
Healthcare’s “Holy Grail” is interoperability. It’s been missing in action while getting lots of notice in planning. But with the ONC’s new urgency to achieve interoperability by 2017 – sooner than envisioned in 2013 – we’re seeing a tough road ahead. It may mean “mountain climbing” over many hills of unreliable data, just to get to a base camp near the top.
ONC former Chief Science Officer, Douglas Fridsma, once quipped in 2013 that the US standard of interoperability is a “modem and a fax machine.”
What’s next?
Our many proprietary U.S. clinical documentation systems, each with data error levels that may not be thoroughly understood, may be asked to lead this vanguard to the “interoperability” summit. Let’s get the data issue right — before the path begins to look like a “bridge too far.” Why not fix the reliability of data and help all patients get better care?
Kyle Silvestro is the Founder and CEO of SyTrue, a business and clinical intelligence platform that turns unstructured (and structured) information into semantically interoperable content that can be leveraged to automate ICD-10 clinical coding. Silvestro is a national thought leader in Natural Language Processing (NLP) and has previously worked with leading healthcare organizations in the utilization and adoption of NLP for meaningful use, clinical research, computer assisted coding, interoperability, semantic search, and other interesting projects.